tensorflow data api with keras (passing tensors to keras model)
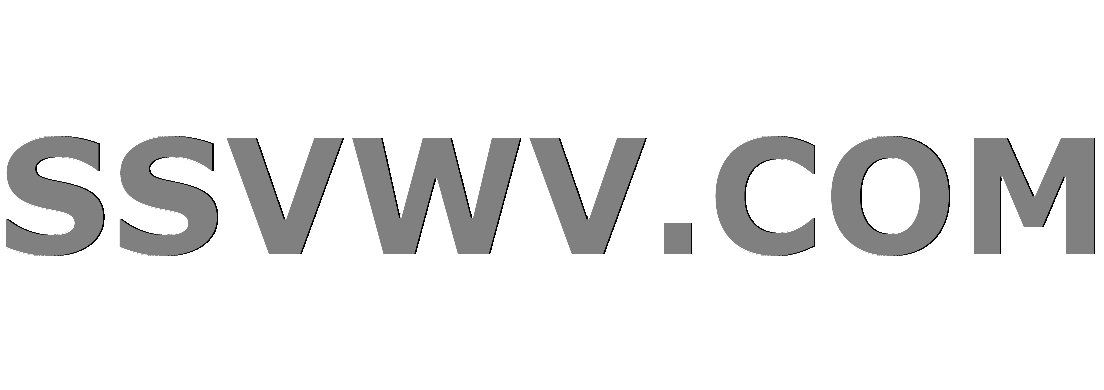
Multi tool use
up vote
0
down vote
favorite
I am trying to train a pretrained keras model on new data. I came across tensorflow's dataset api and I am trying to use it with my old keras model. I understand that tf data api returns tensors, so the data api as well as model should be part of the same graph and the output of the data api should be connected as input to the model. Here is the code
import tensorflow as tf
from data_pipeline import ImageDataGenerator
import os
import keras
from keras.engine import InputLayer
os.environ["CUDA_VISIBLE_DEVICES"]="0"
###################### to check visible devices ###############
from tensorflow.python.client import device_lib
print(device_lib.list_local_devices())
###############################################################
_EPOCHS = 10
_NUM_CLASSES = 2
_BATCH_SIZE = 32
def training_pipeline():
# #############
# Load Dataset
# #############
training_set = ImageDataGenerator(directory="\\in-pdc-sem2\training",
horizontal_flip=True, vertical_flip=True, rescale=True, normalize=True,
color_jitter=True, batch_size=_BATCH_SIZE,
num_cpus=8, epochs=60, output_patch_size=389, validation=False).dataset_pipeline()
testing_set = ImageDataGenerator(directory="\\in-pdc-sem2\training",
horizontal_flip=False, vertical_flip=False, rescale=False, normalize=True,
color_jitter=False, batch_size=_BATCH_SIZE,
num_cpus=8, epochs=60, output_patch_size=389, validation=True).dataset_pipeline()
print(training_set.output_types, training_set.output_shapes)
iterator = tf.data.Iterator.from_structure(training_set.output_types, training_set.output_shapes)#((None, 389, 389, 3), (None)))
train_initializer = iterator.make_initializer(training_set)
validation_initializer = iterator.make_initializer(testing_set)
img, labels = iterator.get_next()
img = img.set_shape((None, 389, 389, 3))
model = baseline_model(img, labels) # keras model defined here
model.summary()
keras.backend.get_session().run(tf.global_variables_initializer())
for epoch in range(_EPOCHS):
# #############
# Train Model
# #############
keras.backend.get_session().run(train_initializer)
model.fit(
steps_per_epoch=1000000 // _BATCH_SIZE,
epochs=1,
# validation_steps=11970 // _BATCH_SIZE,
callbacks=callbacks(),
verbose = 1)
keras.backend.get_session().run(validation_initializer)
loss, acc, cross_entropy = model.evaluate(verbose=1, steps=11970 // 32)
filepath = "./weights/ResNet_16_Best/weights-improvement-Run1-" + str(epoch) + "-" + str(loss) + ".hdf5"
model.save_weights(filepath, overwrite=True)
def baseline_model(input_tensor, labels):
jsonFile = '\\in-pdc-sem2\resnetV4_2Best.json'
weightsFile = '\\in-pdc-sem1\resnetV4_2BestWeightsOnly.hdf5'
with open(jsonFile, "r") as file:
jsonDef = file.read()
from keras.models import model_from_json
model_single = model_from_json(jsonDef)
model_single.load_weights(weightsFile)
model_single.layers[0] = InputLayer(input_tensor=input_tensor, input_shape=(389, 389, 3))
model_single.compile(target_tensors=[labels], loss='categorical_crossentropy', optimizer='Adam', metrics=[keras.metrics.categorical_accuracy])
return model_single
def callbacks():
tensorboard = keras.callbacks.TensorBoard(log_dir='./tensorboard', write_grads=False, write_images=False, histogram_freq=0)
callbacks_list = [tensorboard]
return callbacks_list
if __name__ == '__main__':
training_pipeline()
The "training set" returns image and label tuple, image is a tensor of shape (32, 389, 389, 3), its a batch of 32 images. I verified the shape in a separate script, it is correct. I am defining the input layer of the model using the tensor, and target tensors in the model.compile part.
This is what the model.summary output looks like:
Layer (type) Output Shape Param # Connected to
==================================================================================================
input_1 (InputLayer) (None, 389, 389, 3) 0
__________________________________________________________________________________________________
conv1 (Conv2D) (None, 383, 383, 13) 1924 input_1[0][0]
__________________________________________________________________________________________________
bn_conv1 (BatchNormalization) (None, 383, 383, 13) 52 conv1[0][0]
__________________________________________________________________________________________________
activation_1 (Activation) (None, 383, 383, 13) 0 bn_conv1[0][0]
__________________________________________________________________________________________________
max_pooling2d_1 (MaxPooling2D) (None, 191, 191, 13) 0 activation_1[0][0]
__________________________________________________________________________________________________
res2a_branch2a (Conv2D) (None, 191, 191, 4) 56 max_pooling2d_1[0][0]
__________________________________________________________________________________________________
bn2a_branch2a (BatchNormalizati (None, 191, 191, 4) 16 res2a_branch2a[0][0]
__________________________________________________________________________________________________
activation_2 (Activation) (None, 191, 191, 4) 0 bn2a_branch2a[0][0]
__________________________________________________________________________________________________
res2a_branch2b (Conv2D) (None, 191, 191, 4) 148 activation_2[0][0]
__________________________________________________________________________________________________
bn2a_branch2b (BatchNormalizati (None, 191, 191, 4) 16 res2a_branch2b[0][0]
__________________________________________________________________________________________________
activation_3 (Activation) (None, 191, 191, 4) 0 bn2a_branch2b[0][0]
__________________________________________________________________________________________________
res2a_branch2c (Conv2D) (None, 191, 191, 8) 40 activation_3[0][0]
__________________________________________________________________________________________________
res2a_branch1 (Conv2D) (None, 191, 191, 8) 112 max_pooling2d_1[0][0]
__________________________________________________________________________________________________
bn2a_branch2c (BatchNormalizati (None, 191, 191, 8) 32 res2a_branch2c[0][0]
__________________________________________________________________________________________________
bn2a_branch1 (BatchNormalizatio (None, 191, 191, 8) 32 res2a_branch1[0][0]
__________________________________________________________________________________________________
add_1 (Add) (None, 191, 191, 8) 0 bn2a_branch2c[0][0]
bn2a_branch1[0][0]
__________________________________________________________________________________________________
activation_4 (Activation) (None, 191, 191, 8) 0 add_1[0][0]
__________________________________________________________________________________________________
bn2b_branch2a (BatchNormalizati (None, 191, 191, 8) 32 activation_4[0][0]
__________________________________________________________________________________________________
activation_5 (Activation) (None, 191, 191, 8) 0 bn2b_branch2a[0][0]
__________________________________________________________________________________________________
res2b_branch2b (Conv2D) (None, 191, 191, 4) 292 activation_5[0][0]
__________________________________________________________________________________________________
bn2b_branch2b (BatchNormalizati (None, 191, 191, 4) 16 res2b_branch2b[0][0]
__________________________________________________________________________________________________
activation_6 (Activation) (None, 191, 191, 4) 0 bn2b_branch2b[0][0]
__________________________________________________________________________________________________
res2b_branch2c (Conv2D) (None, 191, 191, 8) 40 activation_6[0][0]
__________________________________________________________________________________________________
add_2 (Add) (None, 191, 191, 8) 0 res2b_branch2c[0][0]
activation_4[0][0]
__________________________________________________________________________________________________
bn2c_branch2a (BatchNormalizati (None, 191, 191, 8) 32 add_2[0][0]
__________________________________________________________________________________________________
activation_7 (Activation) (None, 191, 191, 8) 0 bn2c_branch2a[0][0]
__________________________________________________________________________________________________
res2c_branch2b (Conv2D) (None, 191, 191, 4) 292 activation_7[0][0]
__________________________________________________________________________________________________
bn2c_branch2b (BatchNormalizati (None, 191, 191, 4) 16 res2c_branch2b[0][0]
__________________________________________________________________________________________________
activation_8 (Activation) (None, 191, 191, 4) 0 bn2c_branch2b[0][0]
__________________________________________________________________________________________________
res2c_branch2c (Conv2D) (None, 191, 191, 8) 40 activation_8[0][0]
__________________________________________________________________________________________________
add_3 (Add) (None, 191, 191, 8) 0 res2c_branch2c[0][0]
add_2[0][0]
__________________________________________________________________________________________________
res3a_branch2a (Conv2D) (None, 96, 96, 8) 72 add_3[0][0]
__________________________________________________________________________________________________
bn3a_branch2a (BatchNormalizati (None, 96, 96, 8) 32 res3a_branch2a[0][0]
__________________________________________________________________________________________________
activation_9 (Activation) (None, 96, 96, 8) 0 bn3a_branch2a[0][0]
__________________________________________________________________________________________________
res3a_branch2b (Conv2D) (None, 96, 96, 8) 584 activation_9[0][0]
__________________________________________________________________________________________________
bn3a_branch2b (BatchNormalizati (None, 96, 96, 8) 32 res3a_branch2b[0][0]
__________________________________________________________________________________________________
activation_10 (Activation) (None, 96, 96, 8) 0 bn3a_branch2b[0][0]
__________________________________________________________________________________________________
res3a_branch2c (Conv2D) (None, 96, 96, 16) 144 activation_10[0][0]
__________________________________________________________________________________________________
res3a_branch1 (Conv2D) (None, 96, 96, 16) 144 add_3[0][0]
__________________________________________________________________________________________________
bn3a_branch2c (BatchNormalizati (None, 96, 96, 16) 64 res3a_branch2c[0][0]
__________________________________________________________________________________________________
bn3a_branch1 (BatchNormalizatio (None, 96, 96, 16) 64 res3a_branch1[0][0]
__________________________________________________________________________________________________
add_4 (Add) (None, 96, 96, 16) 0 bn3a_branch2c[0][0]
bn3a_branch1[0][0]
__________________________________________________________________________________________________
activation_11 (Activation) (None, 96, 96, 16) 0 add_4[0][0]
__________________________________________________________________________________________________
bn3b_branch2a (BatchNormalizati (None, 96, 96, 16) 64 activation_11[0][0]
__________________________________________________________________________________________________
activation_12 (Activation) (None, 96, 96, 16) 0 bn3b_branch2a[0][0]
__________________________________________________________________________________________________
res3b_branch2b (Conv2D) (None, 96, 96, 8) 1160 activation_12[0][0]
__________________________________________________________________________________________________
bn3b_branch2b (BatchNormalizati (None, 96, 96, 8) 32 res3b_branch2b[0][0]
__________________________________________________________________________________________________
activation_13 (Activation) (None, 96, 96, 8) 0 bn3b_branch2b[0][0]
__________________________________________________________________________________________________
res3b_branch2c (Conv2D) (None, 96, 96, 16) 144 activation_13[0][0]
__________________________________________________________________________________________________
add_5 (Add) (None, 96, 96, 16) 0 res3b_branch2c[0][0]
activation_11[0][0]
__________________________________________________________________________________________________
res4a_branch2a (Conv2D) (None, 48, 48, 16) 272 add_5[0][0]
__________________________________________________________________________________________________
bn4a_branch2a (BatchNormalizati (None, 48, 48, 16) 64 res4a_branch2a[0][0]
__________________________________________________________________________________________________
activation_14 (Activation) (None, 48, 48, 16) 0 bn4a_branch2a[0][0]
__________________________________________________________________________________________________
res4a_branch2b (Conv2D) (None, 48, 48, 16) 2320 activation_14[0][0]
__________________________________________________________________________________________________
bn4a_branch2b (BatchNormalizati (None, 48, 48, 16) 64 res4a_branch2b[0][0]
__________________________________________________________________________________________________
activation_15 (Activation) (None, 48, 48, 16) 0 bn4a_branch2b[0][0]
__________________________________________________________________________________________________
res4a_branch2c (Conv2D) (None, 48, 48, 64) 1088 activation_15[0][0]
__________________________________________________________________________________________________
res4a_branch1 (Conv2D) (None, 48, 48, 64) 1088 add_5[0][0]
__________________________________________________________________________________________________
bn4a_branch2c (BatchNormalizati (None, 48, 48, 64) 256 res4a_branch2c[0][0]
__________________________________________________________________________________________________
bn4a_branch1 (BatchNormalizatio (None, 48, 48, 64) 256 res4a_branch1[0][0]
__________________________________________________________________________________________________
add_6 (Add) (None, 48, 48, 64) 0 bn4a_branch2c[0][0]
bn4a_branch1[0][0]
__________________________________________________________________________________________________
activation_16 (Activation) (None, 48, 48, 64) 0 add_6[0][0]
__________________________________________________________________________________________________
bn4b_branch2a (BatchNormalizati (None, 48, 48, 64) 256 activation_16[0][0]
__________________________________________________________________________________________________
activation_17 (Activation) (None, 48, 48, 64) 0 bn4b_branch2a[0][0]
__________________________________________________________________________________________________
res4b_branch2b (Conv2D) (None, 48, 48, 16) 9232 activation_17[0][0]
__________________________________________________________________________________________________
bn4b_branch2b (BatchNormalizati (None, 48, 48, 16) 64 res4b_branch2b[0][0]
__________________________________________________________________________________________________
activation_18 (Activation) (None, 48, 48, 16) 0 bn4b_branch2b[0][0]
__________________________________________________________________________________________________
res4b_branch2c (Conv2D) (None, 48, 48, 64) 1088 activation_18[0][0]
__________________________________________________________________________________________________
add_7 (Add) (None, 48, 48, 64) 0 res4b_branch2c[0][0]
activation_16[0][0]
__________________________________________________________________________________________________
res5a_branch2a (Conv2D) (None, 24, 24, 32) 2080 add_7[0][0]
__________________________________________________________________________________________________
bn5a_branch2a (BatchNormalizati (None, 24, 24, 32) 128 res5a_branch2a[0][0]
__________________________________________________________________________________________________
activation_19 (Activation) (None, 24, 24, 32) 0 bn5a_branch2a[0][0]
__________________________________________________________________________________________________
res5a_branch2b (Conv2D) (None, 24, 24, 32) 9248 activation_19[0][0]
__________________________________________________________________________________________________
bn5a_branch2b (BatchNormalizati (None, 24, 24, 32) 128 res5a_branch2b[0][0]
__________________________________________________________________________________________________
activation_20 (Activation) (None, 24, 24, 32) 0 bn5a_branch2b[0][0]
__________________________________________________________________________________________________
res5a_branch2c (Conv2D) (None, 24, 24, 128) 4224 activation_20[0][0]
__________________________________________________________________________________________________
res5a_branch1 (Conv2D) (None, 24, 24, 128) 8320 add_7[0][0]
__________________________________________________________________________________________________
bn5a_branch2c (BatchNormalizati (None, 24, 24, 128) 512 res5a_branch2c[0][0]
__________________________________________________________________________________________________
bn5a_branch1 (BatchNormalizatio (None, 24, 24, 128) 512 res5a_branch1[0][0]
__________________________________________________________________________________________________
add_8 (Add) (None, 24, 24, 128) 0 bn5a_branch2c[0][0]
bn5a_branch1[0][0]
__________________________________________________________________________________________________
activation_21 (Activation) (None, 24, 24, 128) 0 add_8[0][0]
__________________________________________________________________________________________________
res6a_branch2a (Conv2D) (None, 12, 12, 64) 8256 activation_21[0][0]
__________________________________________________________________________________________________
bn6a_branch2a (BatchNormalizati (None, 12, 12, 64) 256 res6a_branch2a[0][0]
__________________________________________________________________________________________________
activation_22 (Activation) (None, 12, 12, 64) 0 bn6a_branch2a[0][0]
__________________________________________________________________________________________________
res6a_branch2b (Conv2D) (None, 12, 12, 64) 36928 activation_22[0][0]
__________________________________________________________________________________________________
bn6a_branch2b (BatchNormalizati (None, 12, 12, 64) 256 res6a_branch2b[0][0]
__________________________________________________________________________________________________
activation_23 (Activation) (None, 12, 12, 64) 0 bn6a_branch2b[0][0]
__________________________________________________________________________________________________
res6a_branch2c (Conv2D) (None, 12, 12, 512) 33280 activation_23[0][0]
__________________________________________________________________________________________________
res6a_branch1 (Conv2D) (None, 12, 12, 512) 66048 activation_21[0][0]
__________________________________________________________________________________________________
bn6a_branch2c (BatchNormalizati (None, 12, 12, 512) 2048 res6a_branch2c[0][0]
__________________________________________________________________________________________________
bn6a_branch1 (BatchNormalizatio (None, 12, 12, 512) 2048 res6a_branch1[0][0]
__________________________________________________________________________________________________
add_9 (Add) (None, 12, 12, 512) 0 bn6a_branch2c[0][0]
bn6a_branch1[0][0]
__________________________________________________________________________________________________
activation_24 (Activation) (None, 12, 12, 512) 0 add_9[0][0]
__________________________________________________________________________________________________
avg_pool (GlobalAveragePooling2 (None, 512) 0 activation_24[0][0]
__________________________________________________________________________________________________
dropout_1 (Dropout) (None, 512) 0 avg_pool[0][0]
__________________________________________________________________________________________________
FC1 (Dense) (None, 1) 513 dropout_1[0][0]
__________________________________________________________________________________________________
activation_25 (Activation) (None, 1) 0 FC1[0][0]
==================================================================================================
Total params: 196,557
Trainable params: 192,867
Non-trainable params: 3,690
Everything looks correct. However When I run the code, I get the following error:
Epoch 1/1
Traceback (most recent call last):
File "C:/Users/ASista162282/Desktop/code/camleyon_17/train.py", line 114, in <module>
training_pipeline()
File "C:/Users/ASista162282/Desktop/code/camleyon_17/train.py", line 71, in training_pipeline
verbose = 1)
File "C:ProgramDataMiniconda3libsite-packageskerasenginetraining.py", line 1705, in fit
validation_steps=validation_steps)
File "C:ProgramDataMiniconda3libsite-packageskerasenginetraining.py", line 1188, in _fit_loop
outs = f(ins)
File "C:ProgramDataMiniconda3libsite-packageskerasbackendtensorflow_backend.py", line 2478, in __call__
**self.session_kwargs)
File "C:ProgramDataMiniconda3libsite-packagestensorflowpythonclientsession.py", line 900, in run
run_metadata_ptr)
File "C:ProgramDataMiniconda3libsite-packagestensorflowpythonclientsession.py", line 1111, in _run
str(subfeed_t.get_shape())))
ValueError: Cannot feed value of shape () for Tensor 'input_1:0', which has shape '(?, 389, 389, 3)'
It doesn't make any sense. I even added the set_shape function before defining the model, and it still shows empty shape. Any help will be really appreciated. Thank you.
tensorflow keras tensorflow-datasets
add a comment |
up vote
0
down vote
favorite
I am trying to train a pretrained keras model on new data. I came across tensorflow's dataset api and I am trying to use it with my old keras model. I understand that tf data api returns tensors, so the data api as well as model should be part of the same graph and the output of the data api should be connected as input to the model. Here is the code
import tensorflow as tf
from data_pipeline import ImageDataGenerator
import os
import keras
from keras.engine import InputLayer
os.environ["CUDA_VISIBLE_DEVICES"]="0"
###################### to check visible devices ###############
from tensorflow.python.client import device_lib
print(device_lib.list_local_devices())
###############################################################
_EPOCHS = 10
_NUM_CLASSES = 2
_BATCH_SIZE = 32
def training_pipeline():
# #############
# Load Dataset
# #############
training_set = ImageDataGenerator(directory="\\in-pdc-sem2\training",
horizontal_flip=True, vertical_flip=True, rescale=True, normalize=True,
color_jitter=True, batch_size=_BATCH_SIZE,
num_cpus=8, epochs=60, output_patch_size=389, validation=False).dataset_pipeline()
testing_set = ImageDataGenerator(directory="\\in-pdc-sem2\training",
horizontal_flip=False, vertical_flip=False, rescale=False, normalize=True,
color_jitter=False, batch_size=_BATCH_SIZE,
num_cpus=8, epochs=60, output_patch_size=389, validation=True).dataset_pipeline()
print(training_set.output_types, training_set.output_shapes)
iterator = tf.data.Iterator.from_structure(training_set.output_types, training_set.output_shapes)#((None, 389, 389, 3), (None)))
train_initializer = iterator.make_initializer(training_set)
validation_initializer = iterator.make_initializer(testing_set)
img, labels = iterator.get_next()
img = img.set_shape((None, 389, 389, 3))
model = baseline_model(img, labels) # keras model defined here
model.summary()
keras.backend.get_session().run(tf.global_variables_initializer())
for epoch in range(_EPOCHS):
# #############
# Train Model
# #############
keras.backend.get_session().run(train_initializer)
model.fit(
steps_per_epoch=1000000 // _BATCH_SIZE,
epochs=1,
# validation_steps=11970 // _BATCH_SIZE,
callbacks=callbacks(),
verbose = 1)
keras.backend.get_session().run(validation_initializer)
loss, acc, cross_entropy = model.evaluate(verbose=1, steps=11970 // 32)
filepath = "./weights/ResNet_16_Best/weights-improvement-Run1-" + str(epoch) + "-" + str(loss) + ".hdf5"
model.save_weights(filepath, overwrite=True)
def baseline_model(input_tensor, labels):
jsonFile = '\\in-pdc-sem2\resnetV4_2Best.json'
weightsFile = '\\in-pdc-sem1\resnetV4_2BestWeightsOnly.hdf5'
with open(jsonFile, "r") as file:
jsonDef = file.read()
from keras.models import model_from_json
model_single = model_from_json(jsonDef)
model_single.load_weights(weightsFile)
model_single.layers[0] = InputLayer(input_tensor=input_tensor, input_shape=(389, 389, 3))
model_single.compile(target_tensors=[labels], loss='categorical_crossentropy', optimizer='Adam', metrics=[keras.metrics.categorical_accuracy])
return model_single
def callbacks():
tensorboard = keras.callbacks.TensorBoard(log_dir='./tensorboard', write_grads=False, write_images=False, histogram_freq=0)
callbacks_list = [tensorboard]
return callbacks_list
if __name__ == '__main__':
training_pipeline()
The "training set" returns image and label tuple, image is a tensor of shape (32, 389, 389, 3), its a batch of 32 images. I verified the shape in a separate script, it is correct. I am defining the input layer of the model using the tensor, and target tensors in the model.compile part.
This is what the model.summary output looks like:
Layer (type) Output Shape Param # Connected to
==================================================================================================
input_1 (InputLayer) (None, 389, 389, 3) 0
__________________________________________________________________________________________________
conv1 (Conv2D) (None, 383, 383, 13) 1924 input_1[0][0]
__________________________________________________________________________________________________
bn_conv1 (BatchNormalization) (None, 383, 383, 13) 52 conv1[0][0]
__________________________________________________________________________________________________
activation_1 (Activation) (None, 383, 383, 13) 0 bn_conv1[0][0]
__________________________________________________________________________________________________
max_pooling2d_1 (MaxPooling2D) (None, 191, 191, 13) 0 activation_1[0][0]
__________________________________________________________________________________________________
res2a_branch2a (Conv2D) (None, 191, 191, 4) 56 max_pooling2d_1[0][0]
__________________________________________________________________________________________________
bn2a_branch2a (BatchNormalizati (None, 191, 191, 4) 16 res2a_branch2a[0][0]
__________________________________________________________________________________________________
activation_2 (Activation) (None, 191, 191, 4) 0 bn2a_branch2a[0][0]
__________________________________________________________________________________________________
res2a_branch2b (Conv2D) (None, 191, 191, 4) 148 activation_2[0][0]
__________________________________________________________________________________________________
bn2a_branch2b (BatchNormalizati (None, 191, 191, 4) 16 res2a_branch2b[0][0]
__________________________________________________________________________________________________
activation_3 (Activation) (None, 191, 191, 4) 0 bn2a_branch2b[0][0]
__________________________________________________________________________________________________
res2a_branch2c (Conv2D) (None, 191, 191, 8) 40 activation_3[0][0]
__________________________________________________________________________________________________
res2a_branch1 (Conv2D) (None, 191, 191, 8) 112 max_pooling2d_1[0][0]
__________________________________________________________________________________________________
bn2a_branch2c (BatchNormalizati (None, 191, 191, 8) 32 res2a_branch2c[0][0]
__________________________________________________________________________________________________
bn2a_branch1 (BatchNormalizatio (None, 191, 191, 8) 32 res2a_branch1[0][0]
__________________________________________________________________________________________________
add_1 (Add) (None, 191, 191, 8) 0 bn2a_branch2c[0][0]
bn2a_branch1[0][0]
__________________________________________________________________________________________________
activation_4 (Activation) (None, 191, 191, 8) 0 add_1[0][0]
__________________________________________________________________________________________________
bn2b_branch2a (BatchNormalizati (None, 191, 191, 8) 32 activation_4[0][0]
__________________________________________________________________________________________________
activation_5 (Activation) (None, 191, 191, 8) 0 bn2b_branch2a[0][0]
__________________________________________________________________________________________________
res2b_branch2b (Conv2D) (None, 191, 191, 4) 292 activation_5[0][0]
__________________________________________________________________________________________________
bn2b_branch2b (BatchNormalizati (None, 191, 191, 4) 16 res2b_branch2b[0][0]
__________________________________________________________________________________________________
activation_6 (Activation) (None, 191, 191, 4) 0 bn2b_branch2b[0][0]
__________________________________________________________________________________________________
res2b_branch2c (Conv2D) (None, 191, 191, 8) 40 activation_6[0][0]
__________________________________________________________________________________________________
add_2 (Add) (None, 191, 191, 8) 0 res2b_branch2c[0][0]
activation_4[0][0]
__________________________________________________________________________________________________
bn2c_branch2a (BatchNormalizati (None, 191, 191, 8) 32 add_2[0][0]
__________________________________________________________________________________________________
activation_7 (Activation) (None, 191, 191, 8) 0 bn2c_branch2a[0][0]
__________________________________________________________________________________________________
res2c_branch2b (Conv2D) (None, 191, 191, 4) 292 activation_7[0][0]
__________________________________________________________________________________________________
bn2c_branch2b (BatchNormalizati (None, 191, 191, 4) 16 res2c_branch2b[0][0]
__________________________________________________________________________________________________
activation_8 (Activation) (None, 191, 191, 4) 0 bn2c_branch2b[0][0]
__________________________________________________________________________________________________
res2c_branch2c (Conv2D) (None, 191, 191, 8) 40 activation_8[0][0]
__________________________________________________________________________________________________
add_3 (Add) (None, 191, 191, 8) 0 res2c_branch2c[0][0]
add_2[0][0]
__________________________________________________________________________________________________
res3a_branch2a (Conv2D) (None, 96, 96, 8) 72 add_3[0][0]
__________________________________________________________________________________________________
bn3a_branch2a (BatchNormalizati (None, 96, 96, 8) 32 res3a_branch2a[0][0]
__________________________________________________________________________________________________
activation_9 (Activation) (None, 96, 96, 8) 0 bn3a_branch2a[0][0]
__________________________________________________________________________________________________
res3a_branch2b (Conv2D) (None, 96, 96, 8) 584 activation_9[0][0]
__________________________________________________________________________________________________
bn3a_branch2b (BatchNormalizati (None, 96, 96, 8) 32 res3a_branch2b[0][0]
__________________________________________________________________________________________________
activation_10 (Activation) (None, 96, 96, 8) 0 bn3a_branch2b[0][0]
__________________________________________________________________________________________________
res3a_branch2c (Conv2D) (None, 96, 96, 16) 144 activation_10[0][0]
__________________________________________________________________________________________________
res3a_branch1 (Conv2D) (None, 96, 96, 16) 144 add_3[0][0]
__________________________________________________________________________________________________
bn3a_branch2c (BatchNormalizati (None, 96, 96, 16) 64 res3a_branch2c[0][0]
__________________________________________________________________________________________________
bn3a_branch1 (BatchNormalizatio (None, 96, 96, 16) 64 res3a_branch1[0][0]
__________________________________________________________________________________________________
add_4 (Add) (None, 96, 96, 16) 0 bn3a_branch2c[0][0]
bn3a_branch1[0][0]
__________________________________________________________________________________________________
activation_11 (Activation) (None, 96, 96, 16) 0 add_4[0][0]
__________________________________________________________________________________________________
bn3b_branch2a (BatchNormalizati (None, 96, 96, 16) 64 activation_11[0][0]
__________________________________________________________________________________________________
activation_12 (Activation) (None, 96, 96, 16) 0 bn3b_branch2a[0][0]
__________________________________________________________________________________________________
res3b_branch2b (Conv2D) (None, 96, 96, 8) 1160 activation_12[0][0]
__________________________________________________________________________________________________
bn3b_branch2b (BatchNormalizati (None, 96, 96, 8) 32 res3b_branch2b[0][0]
__________________________________________________________________________________________________
activation_13 (Activation) (None, 96, 96, 8) 0 bn3b_branch2b[0][0]
__________________________________________________________________________________________________
res3b_branch2c (Conv2D) (None, 96, 96, 16) 144 activation_13[0][0]
__________________________________________________________________________________________________
add_5 (Add) (None, 96, 96, 16) 0 res3b_branch2c[0][0]
activation_11[0][0]
__________________________________________________________________________________________________
res4a_branch2a (Conv2D) (None, 48, 48, 16) 272 add_5[0][0]
__________________________________________________________________________________________________
bn4a_branch2a (BatchNormalizati (None, 48, 48, 16) 64 res4a_branch2a[0][0]
__________________________________________________________________________________________________
activation_14 (Activation) (None, 48, 48, 16) 0 bn4a_branch2a[0][0]
__________________________________________________________________________________________________
res4a_branch2b (Conv2D) (None, 48, 48, 16) 2320 activation_14[0][0]
__________________________________________________________________________________________________
bn4a_branch2b (BatchNormalizati (None, 48, 48, 16) 64 res4a_branch2b[0][0]
__________________________________________________________________________________________________
activation_15 (Activation) (None, 48, 48, 16) 0 bn4a_branch2b[0][0]
__________________________________________________________________________________________________
res4a_branch2c (Conv2D) (None, 48, 48, 64) 1088 activation_15[0][0]
__________________________________________________________________________________________________
res4a_branch1 (Conv2D) (None, 48, 48, 64) 1088 add_5[0][0]
__________________________________________________________________________________________________
bn4a_branch2c (BatchNormalizati (None, 48, 48, 64) 256 res4a_branch2c[0][0]
__________________________________________________________________________________________________
bn4a_branch1 (BatchNormalizatio (None, 48, 48, 64) 256 res4a_branch1[0][0]
__________________________________________________________________________________________________
add_6 (Add) (None, 48, 48, 64) 0 bn4a_branch2c[0][0]
bn4a_branch1[0][0]
__________________________________________________________________________________________________
activation_16 (Activation) (None, 48, 48, 64) 0 add_6[0][0]
__________________________________________________________________________________________________
bn4b_branch2a (BatchNormalizati (None, 48, 48, 64) 256 activation_16[0][0]
__________________________________________________________________________________________________
activation_17 (Activation) (None, 48, 48, 64) 0 bn4b_branch2a[0][0]
__________________________________________________________________________________________________
res4b_branch2b (Conv2D) (None, 48, 48, 16) 9232 activation_17[0][0]
__________________________________________________________________________________________________
bn4b_branch2b (BatchNormalizati (None, 48, 48, 16) 64 res4b_branch2b[0][0]
__________________________________________________________________________________________________
activation_18 (Activation) (None, 48, 48, 16) 0 bn4b_branch2b[0][0]
__________________________________________________________________________________________________
res4b_branch2c (Conv2D) (None, 48, 48, 64) 1088 activation_18[0][0]
__________________________________________________________________________________________________
add_7 (Add) (None, 48, 48, 64) 0 res4b_branch2c[0][0]
activation_16[0][0]
__________________________________________________________________________________________________
res5a_branch2a (Conv2D) (None, 24, 24, 32) 2080 add_7[0][0]
__________________________________________________________________________________________________
bn5a_branch2a (BatchNormalizati (None, 24, 24, 32) 128 res5a_branch2a[0][0]
__________________________________________________________________________________________________
activation_19 (Activation) (None, 24, 24, 32) 0 bn5a_branch2a[0][0]
__________________________________________________________________________________________________
res5a_branch2b (Conv2D) (None, 24, 24, 32) 9248 activation_19[0][0]
__________________________________________________________________________________________________
bn5a_branch2b (BatchNormalizati (None, 24, 24, 32) 128 res5a_branch2b[0][0]
__________________________________________________________________________________________________
activation_20 (Activation) (None, 24, 24, 32) 0 bn5a_branch2b[0][0]
__________________________________________________________________________________________________
res5a_branch2c (Conv2D) (None, 24, 24, 128) 4224 activation_20[0][0]
__________________________________________________________________________________________________
res5a_branch1 (Conv2D) (None, 24, 24, 128) 8320 add_7[0][0]
__________________________________________________________________________________________________
bn5a_branch2c (BatchNormalizati (None, 24, 24, 128) 512 res5a_branch2c[0][0]
__________________________________________________________________________________________________
bn5a_branch1 (BatchNormalizatio (None, 24, 24, 128) 512 res5a_branch1[0][0]
__________________________________________________________________________________________________
add_8 (Add) (None, 24, 24, 128) 0 bn5a_branch2c[0][0]
bn5a_branch1[0][0]
__________________________________________________________________________________________________
activation_21 (Activation) (None, 24, 24, 128) 0 add_8[0][0]
__________________________________________________________________________________________________
res6a_branch2a (Conv2D) (None, 12, 12, 64) 8256 activation_21[0][0]
__________________________________________________________________________________________________
bn6a_branch2a (BatchNormalizati (None, 12, 12, 64) 256 res6a_branch2a[0][0]
__________________________________________________________________________________________________
activation_22 (Activation) (None, 12, 12, 64) 0 bn6a_branch2a[0][0]
__________________________________________________________________________________________________
res6a_branch2b (Conv2D) (None, 12, 12, 64) 36928 activation_22[0][0]
__________________________________________________________________________________________________
bn6a_branch2b (BatchNormalizati (None, 12, 12, 64) 256 res6a_branch2b[0][0]
__________________________________________________________________________________________________
activation_23 (Activation) (None, 12, 12, 64) 0 bn6a_branch2b[0][0]
__________________________________________________________________________________________________
res6a_branch2c (Conv2D) (None, 12, 12, 512) 33280 activation_23[0][0]
__________________________________________________________________________________________________
res6a_branch1 (Conv2D) (None, 12, 12, 512) 66048 activation_21[0][0]
__________________________________________________________________________________________________
bn6a_branch2c (BatchNormalizati (None, 12, 12, 512) 2048 res6a_branch2c[0][0]
__________________________________________________________________________________________________
bn6a_branch1 (BatchNormalizatio (None, 12, 12, 512) 2048 res6a_branch1[0][0]
__________________________________________________________________________________________________
add_9 (Add) (None, 12, 12, 512) 0 bn6a_branch2c[0][0]
bn6a_branch1[0][0]
__________________________________________________________________________________________________
activation_24 (Activation) (None, 12, 12, 512) 0 add_9[0][0]
__________________________________________________________________________________________________
avg_pool (GlobalAveragePooling2 (None, 512) 0 activation_24[0][0]
__________________________________________________________________________________________________
dropout_1 (Dropout) (None, 512) 0 avg_pool[0][0]
__________________________________________________________________________________________________
FC1 (Dense) (None, 1) 513 dropout_1[0][0]
__________________________________________________________________________________________________
activation_25 (Activation) (None, 1) 0 FC1[0][0]
==================================================================================================
Total params: 196,557
Trainable params: 192,867
Non-trainable params: 3,690
Everything looks correct. However When I run the code, I get the following error:
Epoch 1/1
Traceback (most recent call last):
File "C:/Users/ASista162282/Desktop/code/camleyon_17/train.py", line 114, in <module>
training_pipeline()
File "C:/Users/ASista162282/Desktop/code/camleyon_17/train.py", line 71, in training_pipeline
verbose = 1)
File "C:ProgramDataMiniconda3libsite-packageskerasenginetraining.py", line 1705, in fit
validation_steps=validation_steps)
File "C:ProgramDataMiniconda3libsite-packageskerasenginetraining.py", line 1188, in _fit_loop
outs = f(ins)
File "C:ProgramDataMiniconda3libsite-packageskerasbackendtensorflow_backend.py", line 2478, in __call__
**self.session_kwargs)
File "C:ProgramDataMiniconda3libsite-packagestensorflowpythonclientsession.py", line 900, in run
run_metadata_ptr)
File "C:ProgramDataMiniconda3libsite-packagestensorflowpythonclientsession.py", line 1111, in _run
str(subfeed_t.get_shape())))
ValueError: Cannot feed value of shape () for Tensor 'input_1:0', which has shape '(?, 389, 389, 3)'
It doesn't make any sense. I even added the set_shape function before defining the model, and it still shows empty shape. Any help will be really appreciated. Thank you.
tensorflow keras tensorflow-datasets
add a comment |
up vote
0
down vote
favorite
up vote
0
down vote
favorite
I am trying to train a pretrained keras model on new data. I came across tensorflow's dataset api and I am trying to use it with my old keras model. I understand that tf data api returns tensors, so the data api as well as model should be part of the same graph and the output of the data api should be connected as input to the model. Here is the code
import tensorflow as tf
from data_pipeline import ImageDataGenerator
import os
import keras
from keras.engine import InputLayer
os.environ["CUDA_VISIBLE_DEVICES"]="0"
###################### to check visible devices ###############
from tensorflow.python.client import device_lib
print(device_lib.list_local_devices())
###############################################################
_EPOCHS = 10
_NUM_CLASSES = 2
_BATCH_SIZE = 32
def training_pipeline():
# #############
# Load Dataset
# #############
training_set = ImageDataGenerator(directory="\\in-pdc-sem2\training",
horizontal_flip=True, vertical_flip=True, rescale=True, normalize=True,
color_jitter=True, batch_size=_BATCH_SIZE,
num_cpus=8, epochs=60, output_patch_size=389, validation=False).dataset_pipeline()
testing_set = ImageDataGenerator(directory="\\in-pdc-sem2\training",
horizontal_flip=False, vertical_flip=False, rescale=False, normalize=True,
color_jitter=False, batch_size=_BATCH_SIZE,
num_cpus=8, epochs=60, output_patch_size=389, validation=True).dataset_pipeline()
print(training_set.output_types, training_set.output_shapes)
iterator = tf.data.Iterator.from_structure(training_set.output_types, training_set.output_shapes)#((None, 389, 389, 3), (None)))
train_initializer = iterator.make_initializer(training_set)
validation_initializer = iterator.make_initializer(testing_set)
img, labels = iterator.get_next()
img = img.set_shape((None, 389, 389, 3))
model = baseline_model(img, labels) # keras model defined here
model.summary()
keras.backend.get_session().run(tf.global_variables_initializer())
for epoch in range(_EPOCHS):
# #############
# Train Model
# #############
keras.backend.get_session().run(train_initializer)
model.fit(
steps_per_epoch=1000000 // _BATCH_SIZE,
epochs=1,
# validation_steps=11970 // _BATCH_SIZE,
callbacks=callbacks(),
verbose = 1)
keras.backend.get_session().run(validation_initializer)
loss, acc, cross_entropy = model.evaluate(verbose=1, steps=11970 // 32)
filepath = "./weights/ResNet_16_Best/weights-improvement-Run1-" + str(epoch) + "-" + str(loss) + ".hdf5"
model.save_weights(filepath, overwrite=True)
def baseline_model(input_tensor, labels):
jsonFile = '\\in-pdc-sem2\resnetV4_2Best.json'
weightsFile = '\\in-pdc-sem1\resnetV4_2BestWeightsOnly.hdf5'
with open(jsonFile, "r") as file:
jsonDef = file.read()
from keras.models import model_from_json
model_single = model_from_json(jsonDef)
model_single.load_weights(weightsFile)
model_single.layers[0] = InputLayer(input_tensor=input_tensor, input_shape=(389, 389, 3))
model_single.compile(target_tensors=[labels], loss='categorical_crossentropy', optimizer='Adam', metrics=[keras.metrics.categorical_accuracy])
return model_single
def callbacks():
tensorboard = keras.callbacks.TensorBoard(log_dir='./tensorboard', write_grads=False, write_images=False, histogram_freq=0)
callbacks_list = [tensorboard]
return callbacks_list
if __name__ == '__main__':
training_pipeline()
The "training set" returns image and label tuple, image is a tensor of shape (32, 389, 389, 3), its a batch of 32 images. I verified the shape in a separate script, it is correct. I am defining the input layer of the model using the tensor, and target tensors in the model.compile part.
This is what the model.summary output looks like:
Layer (type) Output Shape Param # Connected to
==================================================================================================
input_1 (InputLayer) (None, 389, 389, 3) 0
__________________________________________________________________________________________________
conv1 (Conv2D) (None, 383, 383, 13) 1924 input_1[0][0]
__________________________________________________________________________________________________
bn_conv1 (BatchNormalization) (None, 383, 383, 13) 52 conv1[0][0]
__________________________________________________________________________________________________
activation_1 (Activation) (None, 383, 383, 13) 0 bn_conv1[0][0]
__________________________________________________________________________________________________
max_pooling2d_1 (MaxPooling2D) (None, 191, 191, 13) 0 activation_1[0][0]
__________________________________________________________________________________________________
res2a_branch2a (Conv2D) (None, 191, 191, 4) 56 max_pooling2d_1[0][0]
__________________________________________________________________________________________________
bn2a_branch2a (BatchNormalizati (None, 191, 191, 4) 16 res2a_branch2a[0][0]
__________________________________________________________________________________________________
activation_2 (Activation) (None, 191, 191, 4) 0 bn2a_branch2a[0][0]
__________________________________________________________________________________________________
res2a_branch2b (Conv2D) (None, 191, 191, 4) 148 activation_2[0][0]
__________________________________________________________________________________________________
bn2a_branch2b (BatchNormalizati (None, 191, 191, 4) 16 res2a_branch2b[0][0]
__________________________________________________________________________________________________
activation_3 (Activation) (None, 191, 191, 4) 0 bn2a_branch2b[0][0]
__________________________________________________________________________________________________
res2a_branch2c (Conv2D) (None, 191, 191, 8) 40 activation_3[0][0]
__________________________________________________________________________________________________
res2a_branch1 (Conv2D) (None, 191, 191, 8) 112 max_pooling2d_1[0][0]
__________________________________________________________________________________________________
bn2a_branch2c (BatchNormalizati (None, 191, 191, 8) 32 res2a_branch2c[0][0]
__________________________________________________________________________________________________
bn2a_branch1 (BatchNormalizatio (None, 191, 191, 8) 32 res2a_branch1[0][0]
__________________________________________________________________________________________________
add_1 (Add) (None, 191, 191, 8) 0 bn2a_branch2c[0][0]
bn2a_branch1[0][0]
__________________________________________________________________________________________________
activation_4 (Activation) (None, 191, 191, 8) 0 add_1[0][0]
__________________________________________________________________________________________________
bn2b_branch2a (BatchNormalizati (None, 191, 191, 8) 32 activation_4[0][0]
__________________________________________________________________________________________________
activation_5 (Activation) (None, 191, 191, 8) 0 bn2b_branch2a[0][0]
__________________________________________________________________________________________________
res2b_branch2b (Conv2D) (None, 191, 191, 4) 292 activation_5[0][0]
__________________________________________________________________________________________________
bn2b_branch2b (BatchNormalizati (None, 191, 191, 4) 16 res2b_branch2b[0][0]
__________________________________________________________________________________________________
activation_6 (Activation) (None, 191, 191, 4) 0 bn2b_branch2b[0][0]
__________________________________________________________________________________________________
res2b_branch2c (Conv2D) (None, 191, 191, 8) 40 activation_6[0][0]
__________________________________________________________________________________________________
add_2 (Add) (None, 191, 191, 8) 0 res2b_branch2c[0][0]
activation_4[0][0]
__________________________________________________________________________________________________
bn2c_branch2a (BatchNormalizati (None, 191, 191, 8) 32 add_2[0][0]
__________________________________________________________________________________________________
activation_7 (Activation) (None, 191, 191, 8) 0 bn2c_branch2a[0][0]
__________________________________________________________________________________________________
res2c_branch2b (Conv2D) (None, 191, 191, 4) 292 activation_7[0][0]
__________________________________________________________________________________________________
bn2c_branch2b (BatchNormalizati (None, 191, 191, 4) 16 res2c_branch2b[0][0]
__________________________________________________________________________________________________
activation_8 (Activation) (None, 191, 191, 4) 0 bn2c_branch2b[0][0]
__________________________________________________________________________________________________
res2c_branch2c (Conv2D) (None, 191, 191, 8) 40 activation_8[0][0]
__________________________________________________________________________________________________
add_3 (Add) (None, 191, 191, 8) 0 res2c_branch2c[0][0]
add_2[0][0]
__________________________________________________________________________________________________
res3a_branch2a (Conv2D) (None, 96, 96, 8) 72 add_3[0][0]
__________________________________________________________________________________________________
bn3a_branch2a (BatchNormalizati (None, 96, 96, 8) 32 res3a_branch2a[0][0]
__________________________________________________________________________________________________
activation_9 (Activation) (None, 96, 96, 8) 0 bn3a_branch2a[0][0]
__________________________________________________________________________________________________
res3a_branch2b (Conv2D) (None, 96, 96, 8) 584 activation_9[0][0]
__________________________________________________________________________________________________
bn3a_branch2b (BatchNormalizati (None, 96, 96, 8) 32 res3a_branch2b[0][0]
__________________________________________________________________________________________________
activation_10 (Activation) (None, 96, 96, 8) 0 bn3a_branch2b[0][0]
__________________________________________________________________________________________________
res3a_branch2c (Conv2D) (None, 96, 96, 16) 144 activation_10[0][0]
__________________________________________________________________________________________________
res3a_branch1 (Conv2D) (None, 96, 96, 16) 144 add_3[0][0]
__________________________________________________________________________________________________
bn3a_branch2c (BatchNormalizati (None, 96, 96, 16) 64 res3a_branch2c[0][0]
__________________________________________________________________________________________________
bn3a_branch1 (BatchNormalizatio (None, 96, 96, 16) 64 res3a_branch1[0][0]
__________________________________________________________________________________________________
add_4 (Add) (None, 96, 96, 16) 0 bn3a_branch2c[0][0]
bn3a_branch1[0][0]
__________________________________________________________________________________________________
activation_11 (Activation) (None, 96, 96, 16) 0 add_4[0][0]
__________________________________________________________________________________________________
bn3b_branch2a (BatchNormalizati (None, 96, 96, 16) 64 activation_11[0][0]
__________________________________________________________________________________________________
activation_12 (Activation) (None, 96, 96, 16) 0 bn3b_branch2a[0][0]
__________________________________________________________________________________________________
res3b_branch2b (Conv2D) (None, 96, 96, 8) 1160 activation_12[0][0]
__________________________________________________________________________________________________
bn3b_branch2b (BatchNormalizati (None, 96, 96, 8) 32 res3b_branch2b[0][0]
__________________________________________________________________________________________________
activation_13 (Activation) (None, 96, 96, 8) 0 bn3b_branch2b[0][0]
__________________________________________________________________________________________________
res3b_branch2c (Conv2D) (None, 96, 96, 16) 144 activation_13[0][0]
__________________________________________________________________________________________________
add_5 (Add) (None, 96, 96, 16) 0 res3b_branch2c[0][0]
activation_11[0][0]
__________________________________________________________________________________________________
res4a_branch2a (Conv2D) (None, 48, 48, 16) 272 add_5[0][0]
__________________________________________________________________________________________________
bn4a_branch2a (BatchNormalizati (None, 48, 48, 16) 64 res4a_branch2a[0][0]
__________________________________________________________________________________________________
activation_14 (Activation) (None, 48, 48, 16) 0 bn4a_branch2a[0][0]
__________________________________________________________________________________________________
res4a_branch2b (Conv2D) (None, 48, 48, 16) 2320 activation_14[0][0]
__________________________________________________________________________________________________
bn4a_branch2b (BatchNormalizati (None, 48, 48, 16) 64 res4a_branch2b[0][0]
__________________________________________________________________________________________________
activation_15 (Activation) (None, 48, 48, 16) 0 bn4a_branch2b[0][0]
__________________________________________________________________________________________________
res4a_branch2c (Conv2D) (None, 48, 48, 64) 1088 activation_15[0][0]
__________________________________________________________________________________________________
res4a_branch1 (Conv2D) (None, 48, 48, 64) 1088 add_5[0][0]
__________________________________________________________________________________________________
bn4a_branch2c (BatchNormalizati (None, 48, 48, 64) 256 res4a_branch2c[0][0]
__________________________________________________________________________________________________
bn4a_branch1 (BatchNormalizatio (None, 48, 48, 64) 256 res4a_branch1[0][0]
__________________________________________________________________________________________________
add_6 (Add) (None, 48, 48, 64) 0 bn4a_branch2c[0][0]
bn4a_branch1[0][0]
__________________________________________________________________________________________________
activation_16 (Activation) (None, 48, 48, 64) 0 add_6[0][0]
__________________________________________________________________________________________________
bn4b_branch2a (BatchNormalizati (None, 48, 48, 64) 256 activation_16[0][0]
__________________________________________________________________________________________________
activation_17 (Activation) (None, 48, 48, 64) 0 bn4b_branch2a[0][0]
__________________________________________________________________________________________________
res4b_branch2b (Conv2D) (None, 48, 48, 16) 9232 activation_17[0][0]
__________________________________________________________________________________________________
bn4b_branch2b (BatchNormalizati (None, 48, 48, 16) 64 res4b_branch2b[0][0]
__________________________________________________________________________________________________
activation_18 (Activation) (None, 48, 48, 16) 0 bn4b_branch2b[0][0]
__________________________________________________________________________________________________
res4b_branch2c (Conv2D) (None, 48, 48, 64) 1088 activation_18[0][0]
__________________________________________________________________________________________________
add_7 (Add) (None, 48, 48, 64) 0 res4b_branch2c[0][0]
activation_16[0][0]
__________________________________________________________________________________________________
res5a_branch2a (Conv2D) (None, 24, 24, 32) 2080 add_7[0][0]
__________________________________________________________________________________________________
bn5a_branch2a (BatchNormalizati (None, 24, 24, 32) 128 res5a_branch2a[0][0]
__________________________________________________________________________________________________
activation_19 (Activation) (None, 24, 24, 32) 0 bn5a_branch2a[0][0]
__________________________________________________________________________________________________
res5a_branch2b (Conv2D) (None, 24, 24, 32) 9248 activation_19[0][0]
__________________________________________________________________________________________________
bn5a_branch2b (BatchNormalizati (None, 24, 24, 32) 128 res5a_branch2b[0][0]
__________________________________________________________________________________________________
activation_20 (Activation) (None, 24, 24, 32) 0 bn5a_branch2b[0][0]
__________________________________________________________________________________________________
res5a_branch2c (Conv2D) (None, 24, 24, 128) 4224 activation_20[0][0]
__________________________________________________________________________________________________
res5a_branch1 (Conv2D) (None, 24, 24, 128) 8320 add_7[0][0]
__________________________________________________________________________________________________
bn5a_branch2c (BatchNormalizati (None, 24, 24, 128) 512 res5a_branch2c[0][0]
__________________________________________________________________________________________________
bn5a_branch1 (BatchNormalizatio (None, 24, 24, 128) 512 res5a_branch1[0][0]
__________________________________________________________________________________________________
add_8 (Add) (None, 24, 24, 128) 0 bn5a_branch2c[0][0]
bn5a_branch1[0][0]
__________________________________________________________________________________________________
activation_21 (Activation) (None, 24, 24, 128) 0 add_8[0][0]
__________________________________________________________________________________________________
res6a_branch2a (Conv2D) (None, 12, 12, 64) 8256 activation_21[0][0]
__________________________________________________________________________________________________
bn6a_branch2a (BatchNormalizati (None, 12, 12, 64) 256 res6a_branch2a[0][0]
__________________________________________________________________________________________________
activation_22 (Activation) (None, 12, 12, 64) 0 bn6a_branch2a[0][0]
__________________________________________________________________________________________________
res6a_branch2b (Conv2D) (None, 12, 12, 64) 36928 activation_22[0][0]
__________________________________________________________________________________________________
bn6a_branch2b (BatchNormalizati (None, 12, 12, 64) 256 res6a_branch2b[0][0]
__________________________________________________________________________________________________
activation_23 (Activation) (None, 12, 12, 64) 0 bn6a_branch2b[0][0]
__________________________________________________________________________________________________
res6a_branch2c (Conv2D) (None, 12, 12, 512) 33280 activation_23[0][0]
__________________________________________________________________________________________________
res6a_branch1 (Conv2D) (None, 12, 12, 512) 66048 activation_21[0][0]
__________________________________________________________________________________________________
bn6a_branch2c (BatchNormalizati (None, 12, 12, 512) 2048 res6a_branch2c[0][0]
__________________________________________________________________________________________________
bn6a_branch1 (BatchNormalizatio (None, 12, 12, 512) 2048 res6a_branch1[0][0]
__________________________________________________________________________________________________
add_9 (Add) (None, 12, 12, 512) 0 bn6a_branch2c[0][0]
bn6a_branch1[0][0]
__________________________________________________________________________________________________
activation_24 (Activation) (None, 12, 12, 512) 0 add_9[0][0]
__________________________________________________________________________________________________
avg_pool (GlobalAveragePooling2 (None, 512) 0 activation_24[0][0]
__________________________________________________________________________________________________
dropout_1 (Dropout) (None, 512) 0 avg_pool[0][0]
__________________________________________________________________________________________________
FC1 (Dense) (None, 1) 513 dropout_1[0][0]
__________________________________________________________________________________________________
activation_25 (Activation) (None, 1) 0 FC1[0][0]
==================================================================================================
Total params: 196,557
Trainable params: 192,867
Non-trainable params: 3,690
Everything looks correct. However When I run the code, I get the following error:
Epoch 1/1
Traceback (most recent call last):
File "C:/Users/ASista162282/Desktop/code/camleyon_17/train.py", line 114, in <module>
training_pipeline()
File "C:/Users/ASista162282/Desktop/code/camleyon_17/train.py", line 71, in training_pipeline
verbose = 1)
File "C:ProgramDataMiniconda3libsite-packageskerasenginetraining.py", line 1705, in fit
validation_steps=validation_steps)
File "C:ProgramDataMiniconda3libsite-packageskerasenginetraining.py", line 1188, in _fit_loop
outs = f(ins)
File "C:ProgramDataMiniconda3libsite-packageskerasbackendtensorflow_backend.py", line 2478, in __call__
**self.session_kwargs)
File "C:ProgramDataMiniconda3libsite-packagestensorflowpythonclientsession.py", line 900, in run
run_metadata_ptr)
File "C:ProgramDataMiniconda3libsite-packagestensorflowpythonclientsession.py", line 1111, in _run
str(subfeed_t.get_shape())))
ValueError: Cannot feed value of shape () for Tensor 'input_1:0', which has shape '(?, 389, 389, 3)'
It doesn't make any sense. I even added the set_shape function before defining the model, and it still shows empty shape. Any help will be really appreciated. Thank you.
tensorflow keras tensorflow-datasets
I am trying to train a pretrained keras model on new data. I came across tensorflow's dataset api and I am trying to use it with my old keras model. I understand that tf data api returns tensors, so the data api as well as model should be part of the same graph and the output of the data api should be connected as input to the model. Here is the code
import tensorflow as tf
from data_pipeline import ImageDataGenerator
import os
import keras
from keras.engine import InputLayer
os.environ["CUDA_VISIBLE_DEVICES"]="0"
###################### to check visible devices ###############
from tensorflow.python.client import device_lib
print(device_lib.list_local_devices())
###############################################################
_EPOCHS = 10
_NUM_CLASSES = 2
_BATCH_SIZE = 32
def training_pipeline():
# #############
# Load Dataset
# #############
training_set = ImageDataGenerator(directory="\\in-pdc-sem2\training",
horizontal_flip=True, vertical_flip=True, rescale=True, normalize=True,
color_jitter=True, batch_size=_BATCH_SIZE,
num_cpus=8, epochs=60, output_patch_size=389, validation=False).dataset_pipeline()
testing_set = ImageDataGenerator(directory="\\in-pdc-sem2\training",
horizontal_flip=False, vertical_flip=False, rescale=False, normalize=True,
color_jitter=False, batch_size=_BATCH_SIZE,
num_cpus=8, epochs=60, output_patch_size=389, validation=True).dataset_pipeline()
print(training_set.output_types, training_set.output_shapes)
iterator = tf.data.Iterator.from_structure(training_set.output_types, training_set.output_shapes)#((None, 389, 389, 3), (None)))
train_initializer = iterator.make_initializer(training_set)
validation_initializer = iterator.make_initializer(testing_set)
img, labels = iterator.get_next()
img = img.set_shape((None, 389, 389, 3))
model = baseline_model(img, labels) # keras model defined here
model.summary()
keras.backend.get_session().run(tf.global_variables_initializer())
for epoch in range(_EPOCHS):
# #############
# Train Model
# #############
keras.backend.get_session().run(train_initializer)
model.fit(
steps_per_epoch=1000000 // _BATCH_SIZE,
epochs=1,
# validation_steps=11970 // _BATCH_SIZE,
callbacks=callbacks(),
verbose = 1)
keras.backend.get_session().run(validation_initializer)
loss, acc, cross_entropy = model.evaluate(verbose=1, steps=11970 // 32)
filepath = "./weights/ResNet_16_Best/weights-improvement-Run1-" + str(epoch) + "-" + str(loss) + ".hdf5"
model.save_weights(filepath, overwrite=True)
def baseline_model(input_tensor, labels):
jsonFile = '\\in-pdc-sem2\resnetV4_2Best.json'
weightsFile = '\\in-pdc-sem1\resnetV4_2BestWeightsOnly.hdf5'
with open(jsonFile, "r") as file:
jsonDef = file.read()
from keras.models import model_from_json
model_single = model_from_json(jsonDef)
model_single.load_weights(weightsFile)
model_single.layers[0] = InputLayer(input_tensor=input_tensor, input_shape=(389, 389, 3))
model_single.compile(target_tensors=[labels], loss='categorical_crossentropy', optimizer='Adam', metrics=[keras.metrics.categorical_accuracy])
return model_single
def callbacks():
tensorboard = keras.callbacks.TensorBoard(log_dir='./tensorboard', write_grads=False, write_images=False, histogram_freq=0)
callbacks_list = [tensorboard]
return callbacks_list
if __name__ == '__main__':
training_pipeline()
The "training set" returns image and label tuple, image is a tensor of shape (32, 389, 389, 3), its a batch of 32 images. I verified the shape in a separate script, it is correct. I am defining the input layer of the model using the tensor, and target tensors in the model.compile part.
This is what the model.summary output looks like:
Layer (type) Output Shape Param # Connected to
==================================================================================================
input_1 (InputLayer) (None, 389, 389, 3) 0
__________________________________________________________________________________________________
conv1 (Conv2D) (None, 383, 383, 13) 1924 input_1[0][0]
__________________________________________________________________________________________________
bn_conv1 (BatchNormalization) (None, 383, 383, 13) 52 conv1[0][0]
__________________________________________________________________________________________________
activation_1 (Activation) (None, 383, 383, 13) 0 bn_conv1[0][0]
__________________________________________________________________________________________________
max_pooling2d_1 (MaxPooling2D) (None, 191, 191, 13) 0 activation_1[0][0]
__________________________________________________________________________________________________
res2a_branch2a (Conv2D) (None, 191, 191, 4) 56 max_pooling2d_1[0][0]
__________________________________________________________________________________________________
bn2a_branch2a (BatchNormalizati (None, 191, 191, 4) 16 res2a_branch2a[0][0]
__________________________________________________________________________________________________
activation_2 (Activation) (None, 191, 191, 4) 0 bn2a_branch2a[0][0]
__________________________________________________________________________________________________
res2a_branch2b (Conv2D) (None, 191, 191, 4) 148 activation_2[0][0]
__________________________________________________________________________________________________
bn2a_branch2b (BatchNormalizati (None, 191, 191, 4) 16 res2a_branch2b[0][0]
__________________________________________________________________________________________________
activation_3 (Activation) (None, 191, 191, 4) 0 bn2a_branch2b[0][0]
__________________________________________________________________________________________________
res2a_branch2c (Conv2D) (None, 191, 191, 8) 40 activation_3[0][0]
__________________________________________________________________________________________________
res2a_branch1 (Conv2D) (None, 191, 191, 8) 112 max_pooling2d_1[0][0]
__________________________________________________________________________________________________
bn2a_branch2c (BatchNormalizati (None, 191, 191, 8) 32 res2a_branch2c[0][0]
__________________________________________________________________________________________________
bn2a_branch1 (BatchNormalizatio (None, 191, 191, 8) 32 res2a_branch1[0][0]
__________________________________________________________________________________________________
add_1 (Add) (None, 191, 191, 8) 0 bn2a_branch2c[0][0]
bn2a_branch1[0][0]
__________________________________________________________________________________________________
activation_4 (Activation) (None, 191, 191, 8) 0 add_1[0][0]
__________________________________________________________________________________________________
bn2b_branch2a (BatchNormalizati (None, 191, 191, 8) 32 activation_4[0][0]
__________________________________________________________________________________________________
activation_5 (Activation) (None, 191, 191, 8) 0 bn2b_branch2a[0][0]
__________________________________________________________________________________________________
res2b_branch2b (Conv2D) (None, 191, 191, 4) 292 activation_5[0][0]
__________________________________________________________________________________________________
bn2b_branch2b (BatchNormalizati (None, 191, 191, 4) 16 res2b_branch2b[0][0]
__________________________________________________________________________________________________
activation_6 (Activation) (None, 191, 191, 4) 0 bn2b_branch2b[0][0]
__________________________________________________________________________________________________
res2b_branch2c (Conv2D) (None, 191, 191, 8) 40 activation_6[0][0]
__________________________________________________________________________________________________
add_2 (Add) (None, 191, 191, 8) 0 res2b_branch2c[0][0]
activation_4[0][0]
__________________________________________________________________________________________________
bn2c_branch2a (BatchNormalizati (None, 191, 191, 8) 32 add_2[0][0]
__________________________________________________________________________________________________
activation_7 (Activation) (None, 191, 191, 8) 0 bn2c_branch2a[0][0]
__________________________________________________________________________________________________
res2c_branch2b (Conv2D) (None, 191, 191, 4) 292 activation_7[0][0]
__________________________________________________________________________________________________
bn2c_branch2b (BatchNormalizati (None, 191, 191, 4) 16 res2c_branch2b[0][0]
__________________________________________________________________________________________________
activation_8 (Activation) (None, 191, 191, 4) 0 bn2c_branch2b[0][0]
__________________________________________________________________________________________________
res2c_branch2c (Conv2D) (None, 191, 191, 8) 40 activation_8[0][0]
__________________________________________________________________________________________________
add_3 (Add) (None, 191, 191, 8) 0 res2c_branch2c[0][0]
add_2[0][0]
__________________________________________________________________________________________________
res3a_branch2a (Conv2D) (None, 96, 96, 8) 72 add_3[0][0]
__________________________________________________________________________________________________
bn3a_branch2a (BatchNormalizati (None, 96, 96, 8) 32 res3a_branch2a[0][0]
__________________________________________________________________________________________________
activation_9 (Activation) (None, 96, 96, 8) 0 bn3a_branch2a[0][0]
__________________________________________________________________________________________________
res3a_branch2b (Conv2D) (None, 96, 96, 8) 584 activation_9[0][0]
__________________________________________________________________________________________________
bn3a_branch2b (BatchNormalizati (None, 96, 96, 8) 32 res3a_branch2b[0][0]
__________________________________________________________________________________________________
activation_10 (Activation) (None, 96, 96, 8) 0 bn3a_branch2b[0][0]
__________________________________________________________________________________________________
res3a_branch2c (Conv2D) (None, 96, 96, 16) 144 activation_10[0][0]
__________________________________________________________________________________________________
res3a_branch1 (Conv2D) (None, 96, 96, 16) 144 add_3[0][0]
__________________________________________________________________________________________________
bn3a_branch2c (BatchNormalizati (None, 96, 96, 16) 64 res3a_branch2c[0][0]
__________________________________________________________________________________________________
bn3a_branch1 (BatchNormalizatio (None, 96, 96, 16) 64 res3a_branch1[0][0]
__________________________________________________________________________________________________
add_4 (Add) (None, 96, 96, 16) 0 bn3a_branch2c[0][0]
bn3a_branch1[0][0]
__________________________________________________________________________________________________
activation_11 (Activation) (None, 96, 96, 16) 0 add_4[0][0]
__________________________________________________________________________________________________
bn3b_branch2a (BatchNormalizati (None, 96, 96, 16) 64 activation_11[0][0]
__________________________________________________________________________________________________
activation_12 (Activation) (None, 96, 96, 16) 0 bn3b_branch2a[0][0]
__________________________________________________________________________________________________
res3b_branch2b (Conv2D) (None, 96, 96, 8) 1160 activation_12[0][0]
__________________________________________________________________________________________________
bn3b_branch2b (BatchNormalizati (None, 96, 96, 8) 32 res3b_branch2b[0][0]
__________________________________________________________________________________________________
activation_13 (Activation) (None, 96, 96, 8) 0 bn3b_branch2b[0][0]
__________________________________________________________________________________________________
res3b_branch2c (Conv2D) (None, 96, 96, 16) 144 activation_13[0][0]
__________________________________________________________________________________________________
add_5 (Add) (None, 96, 96, 16) 0 res3b_branch2c[0][0]
activation_11[0][0]
__________________________________________________________________________________________________
res4a_branch2a (Conv2D) (None, 48, 48, 16) 272 add_5[0][0]
__________________________________________________________________________________________________
bn4a_branch2a (BatchNormalizati (None, 48, 48, 16) 64 res4a_branch2a[0][0]
__________________________________________________________________________________________________
activation_14 (Activation) (None, 48, 48, 16) 0 bn4a_branch2a[0][0]
__________________________________________________________________________________________________
res4a_branch2b (Conv2D) (None, 48, 48, 16) 2320 activation_14[0][0]
__________________________________________________________________________________________________
bn4a_branch2b (BatchNormalizati (None, 48, 48, 16) 64 res4a_branch2b[0][0]
__________________________________________________________________________________________________
activation_15 (Activation) (None, 48, 48, 16) 0 bn4a_branch2b[0][0]
__________________________________________________________________________________________________
res4a_branch2c (Conv2D) (None, 48, 48, 64) 1088 activation_15[0][0]
__________________________________________________________________________________________________
res4a_branch1 (Conv2D) (None, 48, 48, 64) 1088 add_5[0][0]
__________________________________________________________________________________________________
bn4a_branch2c (BatchNormalizati (None, 48, 48, 64) 256 res4a_branch2c[0][0]
__________________________________________________________________________________________________
bn4a_branch1 (BatchNormalizatio (None, 48, 48, 64) 256 res4a_branch1[0][0]
__________________________________________________________________________________________________
add_6 (Add) (None, 48, 48, 64) 0 bn4a_branch2c[0][0]
bn4a_branch1[0][0]
__________________________________________________________________________________________________
activation_16 (Activation) (None, 48, 48, 64) 0 add_6[0][0]
__________________________________________________________________________________________________
bn4b_branch2a (BatchNormalizati (None, 48, 48, 64) 256 activation_16[0][0]
__________________________________________________________________________________________________
activation_17 (Activation) (None, 48, 48, 64) 0 bn4b_branch2a[0][0]
__________________________________________________________________________________________________
res4b_branch2b (Conv2D) (None, 48, 48, 16) 9232 activation_17[0][0]
__________________________________________________________________________________________________
bn4b_branch2b (BatchNormalizati (None, 48, 48, 16) 64 res4b_branch2b[0][0]
__________________________________________________________________________________________________
activation_18 (Activation) (None, 48, 48, 16) 0 bn4b_branch2b[0][0]
__________________________________________________________________________________________________
res4b_branch2c (Conv2D) (None, 48, 48, 64) 1088 activation_18[0][0]
__________________________________________________________________________________________________
add_7 (Add) (None, 48, 48, 64) 0 res4b_branch2c[0][0]
activation_16[0][0]
__________________________________________________________________________________________________
res5a_branch2a (Conv2D) (None, 24, 24, 32) 2080 add_7[0][0]
__________________________________________________________________________________________________
bn5a_branch2a (BatchNormalizati (None, 24, 24, 32) 128 res5a_branch2a[0][0]
__________________________________________________________________________________________________
activation_19 (Activation) (None, 24, 24, 32) 0 bn5a_branch2a[0][0]
__________________________________________________________________________________________________
res5a_branch2b (Conv2D) (None, 24, 24, 32) 9248 activation_19[0][0]
__________________________________________________________________________________________________
bn5a_branch2b (BatchNormalizati (None, 24, 24, 32) 128 res5a_branch2b[0][0]
__________________________________________________________________________________________________
activation_20 (Activation) (None, 24, 24, 32) 0 bn5a_branch2b[0][0]
__________________________________________________________________________________________________
res5a_branch2c (Conv2D) (None, 24, 24, 128) 4224 activation_20[0][0]
__________________________________________________________________________________________________
res5a_branch1 (Conv2D) (None, 24, 24, 128) 8320 add_7[0][0]
__________________________________________________________________________________________________
bn5a_branch2c (BatchNormalizati (None, 24, 24, 128) 512 res5a_branch2c[0][0]
__________________________________________________________________________________________________
bn5a_branch1 (BatchNormalizatio (None, 24, 24, 128) 512 res5a_branch1[0][0]
__________________________________________________________________________________________________
add_8 (Add) (None, 24, 24, 128) 0 bn5a_branch2c[0][0]
bn5a_branch1[0][0]
__________________________________________________________________________________________________
activation_21 (Activation) (None, 24, 24, 128) 0 add_8[0][0]
__________________________________________________________________________________________________
res6a_branch2a (Conv2D) (None, 12, 12, 64) 8256 activation_21[0][0]
__________________________________________________________________________________________________
bn6a_branch2a (BatchNormalizati (None, 12, 12, 64) 256 res6a_branch2a[0][0]
__________________________________________________________________________________________________
activation_22 (Activation) (None, 12, 12, 64) 0 bn6a_branch2a[0][0]
__________________________________________________________________________________________________
res6a_branch2b (Conv2D) (None, 12, 12, 64) 36928 activation_22[0][0]
__________________________________________________________________________________________________
bn6a_branch2b (BatchNormalizati (None, 12, 12, 64) 256 res6a_branch2b[0][0]
__________________________________________________________________________________________________
activation_23 (Activation) (None, 12, 12, 64) 0 bn6a_branch2b[0][0]
__________________________________________________________________________________________________
res6a_branch2c (Conv2D) (None, 12, 12, 512) 33280 activation_23[0][0]
__________________________________________________________________________________________________
res6a_branch1 (Conv2D) (None, 12, 12, 512) 66048 activation_21[0][0]
__________________________________________________________________________________________________
bn6a_branch2c (BatchNormalizati (None, 12, 12, 512) 2048 res6a_branch2c[0][0]
__________________________________________________________________________________________________
bn6a_branch1 (BatchNormalizatio (None, 12, 12, 512) 2048 res6a_branch1[0][0]
__________________________________________________________________________________________________
add_9 (Add) (None, 12, 12, 512) 0 bn6a_branch2c[0][0]
bn6a_branch1[0][0]
__________________________________________________________________________________________________
activation_24 (Activation) (None, 12, 12, 512) 0 add_9[0][0]
__________________________________________________________________________________________________
avg_pool (GlobalAveragePooling2 (None, 512) 0 activation_24[0][0]
__________________________________________________________________________________________________
dropout_1 (Dropout) (None, 512) 0 avg_pool[0][0]
__________________________________________________________________________________________________
FC1 (Dense) (None, 1) 513 dropout_1[0][0]
__________________________________________________________________________________________________
activation_25 (Activation) (None, 1) 0 FC1[0][0]
==================================================================================================
Total params: 196,557
Trainable params: 192,867
Non-trainable params: 3,690
Everything looks correct. However When I run the code, I get the following error:
Epoch 1/1
Traceback (most recent call last):
File "C:/Users/ASista162282/Desktop/code/camleyon_17/train.py", line 114, in <module>
training_pipeline()
File "C:/Users/ASista162282/Desktop/code/camleyon_17/train.py", line 71, in training_pipeline
verbose = 1)
File "C:ProgramDataMiniconda3libsite-packageskerasenginetraining.py", line 1705, in fit
validation_steps=validation_steps)
File "C:ProgramDataMiniconda3libsite-packageskerasenginetraining.py", line 1188, in _fit_loop
outs = f(ins)
File "C:ProgramDataMiniconda3libsite-packageskerasbackendtensorflow_backend.py", line 2478, in __call__
**self.session_kwargs)
File "C:ProgramDataMiniconda3libsite-packagestensorflowpythonclientsession.py", line 900, in run
run_metadata_ptr)
File "C:ProgramDataMiniconda3libsite-packagestensorflowpythonclientsession.py", line 1111, in _run
str(subfeed_t.get_shape())))
ValueError: Cannot feed value of shape () for Tensor 'input_1:0', which has shape '(?, 389, 389, 3)'
It doesn't make any sense. I even added the set_shape function before defining the model, and it still shows empty shape. Any help will be really appreciated. Thank you.
tensorflow keras tensorflow-datasets
tensorflow keras tensorflow-datasets
edited Nov 12 at 10:47
asked Nov 12 at 10:27


aditya sista
427
427
add a comment |
add a comment |
1 Answer
1
active
oldest
votes
up vote
1
down vote
The way you are replacing the input layer doesn't seem to connect the new layer correctly. Try replacing this:
model_single.layers[0] = InputLayer(input_tensor=input_tensor, input_shape=(389, 389, 3))
with this:
from keras.models import Model
model_single.layers.pop(0)
new_input = InputLayer(input_tensor=input_tensor, input_shape=(389, 389, 3))
new_output = model_single(new_input)
model_single = Model(new_input, new_output)
I did that, I am getting the following error: ValueError: Layer resnetV4.2 was called with an input that isn't a symbolic tensor. Received type: <class 'keras.engine.topology.InputLayer'>
– aditya sista
Nov 13 at 7:19
I added another line to my answer (see linemodel_single.layers.pop(0)
) to remove the old input layer that was missing in my original post. This might do it now
– Tadej Magajna
Nov 13 at 9:58
1
That didnt work either. But for some reason using keras.layers.Input worked, rather than InputLayer. No idea why.
– aditya sista
Nov 25 at 21:11
add a comment |
Your Answer
StackExchange.ifUsing("editor", function () {
StackExchange.using("externalEditor", function () {
StackExchange.using("snippets", function () {
StackExchange.snippets.init();
});
});
}, "code-snippets");
StackExchange.ready(function() {
var channelOptions = {
tags: "".split(" "),
id: "1"
};
initTagRenderer("".split(" "), "".split(" "), channelOptions);
StackExchange.using("externalEditor", function() {
// Have to fire editor after snippets, if snippets enabled
if (StackExchange.settings.snippets.snippetsEnabled) {
StackExchange.using("snippets", function() {
createEditor();
});
}
else {
createEditor();
}
});
function createEditor() {
StackExchange.prepareEditor({
heartbeatType: 'answer',
convertImagesToLinks: true,
noModals: true,
showLowRepImageUploadWarning: true,
reputationToPostImages: 10,
bindNavPrevention: true,
postfix: "",
imageUploader: {
brandingHtml: "Powered by u003ca class="icon-imgur-white" href="https://imgur.com/"u003eu003c/au003e",
contentPolicyHtml: "User contributions licensed under u003ca href="https://creativecommons.org/licenses/by-sa/3.0/"u003ecc by-sa 3.0 with attribution requiredu003c/au003e u003ca href="https://stackoverflow.com/legal/content-policy"u003e(content policy)u003c/au003e",
allowUrls: true
},
onDemand: true,
discardSelector: ".discard-answer"
,immediatelyShowMarkdownHelp:true
});
}
});
Sign up or log in
StackExchange.ready(function () {
StackExchange.helpers.onClickDraftSave('#login-link');
});
Sign up using Google
Sign up using Facebook
Sign up using Email and Password
Post as a guest
Required, but never shown
StackExchange.ready(
function () {
StackExchange.openid.initPostLogin('.new-post-login', 'https%3a%2f%2fstackoverflow.com%2fquestions%2f53260221%2ftensorflow-data-api-with-keras-passing-tensors-to-keras-model%23new-answer', 'question_page');
}
);
Post as a guest
Required, but never shown
1 Answer
1
active
oldest
votes
1 Answer
1
active
oldest
votes
active
oldest
votes
active
oldest
votes
up vote
1
down vote
The way you are replacing the input layer doesn't seem to connect the new layer correctly. Try replacing this:
model_single.layers[0] = InputLayer(input_tensor=input_tensor, input_shape=(389, 389, 3))
with this:
from keras.models import Model
model_single.layers.pop(0)
new_input = InputLayer(input_tensor=input_tensor, input_shape=(389, 389, 3))
new_output = model_single(new_input)
model_single = Model(new_input, new_output)
I did that, I am getting the following error: ValueError: Layer resnetV4.2 was called with an input that isn't a symbolic tensor. Received type: <class 'keras.engine.topology.InputLayer'>
– aditya sista
Nov 13 at 7:19
I added another line to my answer (see linemodel_single.layers.pop(0)
) to remove the old input layer that was missing in my original post. This might do it now
– Tadej Magajna
Nov 13 at 9:58
1
That didnt work either. But for some reason using keras.layers.Input worked, rather than InputLayer. No idea why.
– aditya sista
Nov 25 at 21:11
add a comment |
up vote
1
down vote
The way you are replacing the input layer doesn't seem to connect the new layer correctly. Try replacing this:
model_single.layers[0] = InputLayer(input_tensor=input_tensor, input_shape=(389, 389, 3))
with this:
from keras.models import Model
model_single.layers.pop(0)
new_input = InputLayer(input_tensor=input_tensor, input_shape=(389, 389, 3))
new_output = model_single(new_input)
model_single = Model(new_input, new_output)
I did that, I am getting the following error: ValueError: Layer resnetV4.2 was called with an input that isn't a symbolic tensor. Received type: <class 'keras.engine.topology.InputLayer'>
– aditya sista
Nov 13 at 7:19
I added another line to my answer (see linemodel_single.layers.pop(0)
) to remove the old input layer that was missing in my original post. This might do it now
– Tadej Magajna
Nov 13 at 9:58
1
That didnt work either. But for some reason using keras.layers.Input worked, rather than InputLayer. No idea why.
– aditya sista
Nov 25 at 21:11
add a comment |
up vote
1
down vote
up vote
1
down vote
The way you are replacing the input layer doesn't seem to connect the new layer correctly. Try replacing this:
model_single.layers[0] = InputLayer(input_tensor=input_tensor, input_shape=(389, 389, 3))
with this:
from keras.models import Model
model_single.layers.pop(0)
new_input = InputLayer(input_tensor=input_tensor, input_shape=(389, 389, 3))
new_output = model_single(new_input)
model_single = Model(new_input, new_output)
The way you are replacing the input layer doesn't seem to connect the new layer correctly. Try replacing this:
model_single.layers[0] = InputLayer(input_tensor=input_tensor, input_shape=(389, 389, 3))
with this:
from keras.models import Model
model_single.layers.pop(0)
new_input = InputLayer(input_tensor=input_tensor, input_shape=(389, 389, 3))
new_output = model_single(new_input)
model_single = Model(new_input, new_output)
edited Nov 13 at 9:56
answered Nov 12 at 13:18
Tadej Magajna
1,1001232
1,1001232
I did that, I am getting the following error: ValueError: Layer resnetV4.2 was called with an input that isn't a symbolic tensor. Received type: <class 'keras.engine.topology.InputLayer'>
– aditya sista
Nov 13 at 7:19
I added another line to my answer (see linemodel_single.layers.pop(0)
) to remove the old input layer that was missing in my original post. This might do it now
– Tadej Magajna
Nov 13 at 9:58
1
That didnt work either. But for some reason using keras.layers.Input worked, rather than InputLayer. No idea why.
– aditya sista
Nov 25 at 21:11
add a comment |
I did that, I am getting the following error: ValueError: Layer resnetV4.2 was called with an input that isn't a symbolic tensor. Received type: <class 'keras.engine.topology.InputLayer'>
– aditya sista
Nov 13 at 7:19
I added another line to my answer (see linemodel_single.layers.pop(0)
) to remove the old input layer that was missing in my original post. This might do it now
– Tadej Magajna
Nov 13 at 9:58
1
That didnt work either. But for some reason using keras.layers.Input worked, rather than InputLayer. No idea why.
– aditya sista
Nov 25 at 21:11
I did that, I am getting the following error: ValueError: Layer resnetV4.2 was called with an input that isn't a symbolic tensor. Received type: <class 'keras.engine.topology.InputLayer'>
– aditya sista
Nov 13 at 7:19
I did that, I am getting the following error: ValueError: Layer resnetV4.2 was called with an input that isn't a symbolic tensor. Received type: <class 'keras.engine.topology.InputLayer'>
– aditya sista
Nov 13 at 7:19
I added another line to my answer (see line
model_single.layers.pop(0)
) to remove the old input layer that was missing in my original post. This might do it now– Tadej Magajna
Nov 13 at 9:58
I added another line to my answer (see line
model_single.layers.pop(0)
) to remove the old input layer that was missing in my original post. This might do it now– Tadej Magajna
Nov 13 at 9:58
1
1
That didnt work either. But for some reason using keras.layers.Input worked, rather than InputLayer. No idea why.
– aditya sista
Nov 25 at 21:11
That didnt work either. But for some reason using keras.layers.Input worked, rather than InputLayer. No idea why.
– aditya sista
Nov 25 at 21:11
add a comment |
Thanks for contributing an answer to Stack Overflow!
- Please be sure to answer the question. Provide details and share your research!
But avoid …
- Asking for help, clarification, or responding to other answers.
- Making statements based on opinion; back them up with references or personal experience.
To learn more, see our tips on writing great answers.
Some of your past answers have not been well-received, and you're in danger of being blocked from answering.
Please pay close attention to the following guidance:
- Please be sure to answer the question. Provide details and share your research!
But avoid …
- Asking for help, clarification, or responding to other answers.
- Making statements based on opinion; back them up with references or personal experience.
To learn more, see our tips on writing great answers.
Sign up or log in
StackExchange.ready(function () {
StackExchange.helpers.onClickDraftSave('#login-link');
});
Sign up using Google
Sign up using Facebook
Sign up using Email and Password
Post as a guest
Required, but never shown
StackExchange.ready(
function () {
StackExchange.openid.initPostLogin('.new-post-login', 'https%3a%2f%2fstackoverflow.com%2fquestions%2f53260221%2ftensorflow-data-api-with-keras-passing-tensors-to-keras-model%23new-answer', 'question_page');
}
);
Post as a guest
Required, but never shown
Sign up or log in
StackExchange.ready(function () {
StackExchange.helpers.onClickDraftSave('#login-link');
});
Sign up using Google
Sign up using Facebook
Sign up using Email and Password
Post as a guest
Required, but never shown
Sign up or log in
StackExchange.ready(function () {
StackExchange.helpers.onClickDraftSave('#login-link');
});
Sign up using Google
Sign up using Facebook
Sign up using Email and Password
Post as a guest
Required, but never shown
Sign up or log in
StackExchange.ready(function () {
StackExchange.helpers.onClickDraftSave('#login-link');
});
Sign up using Google
Sign up using Facebook
Sign up using Email and Password
Sign up using Google
Sign up using Facebook
Sign up using Email and Password
Post as a guest
Required, but never shown
Required, but never shown
Required, but never shown
Required, but never shown
Required, but never shown
Required, but never shown
Required, but never shown
Required, but never shown
Required, but never shown
I,k3AMH1 3vggl3Cv1n9ghB dp3Twl4gOdS xf6EBDqrF d81ycvd HGAUZ,1P933hmg20xEA