Bin packing with grouping
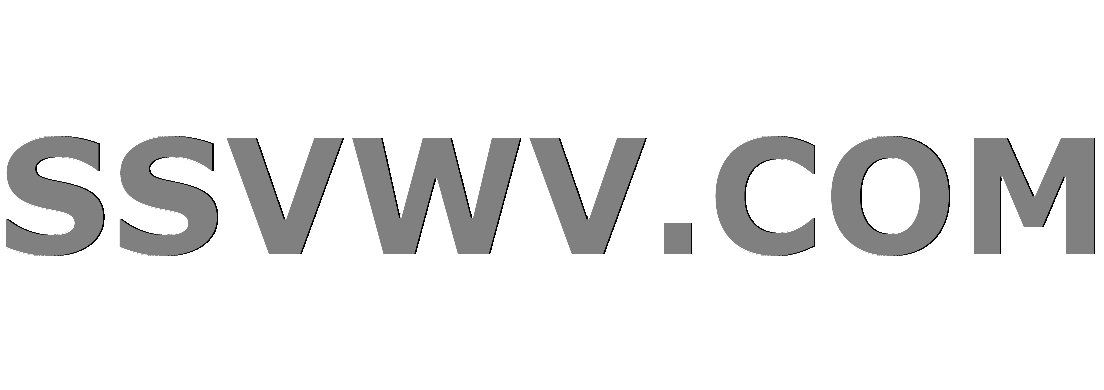
Multi tool use
I have a list of products (i), each with given weight and volume. The optimization goes in two steps, of which I have been unable to solve the second step.
First optimization: minimize number of bins used (solved)
Minimize number of bins used to pack list of products. I have fixed bins with with maximum volume and weight constraints. Python code:
prob = pp.LpProblem("algorithm",pp.LpMinimize) #pp=pulp solver
Y_max=10 #bins will not exceed this number
#Y_min = minimum number of bins (calculated)
# j = index of jth bin
# i = index of ith product
w=weights #list of weights w_i for product i
v=volumes #list of volumes v_i for product i
W=W_max #maximum weight of a bin
V=V_max #maximum volume of a bin
#len(order) = number of products
x=pp.LpVariable.dicts("x_i,j", ((i, j) for i in range(len(order)) for j in range(Y_max)),0,1,pp.LpBinary) #variable indicating if product i is placed in bin j
y=pp.LpVariable.dicts("y_j", ((j,0) for j in range(Y_max)),0,1,pp.LpBinary) #variable indicating if bin j is used or unused
Y=pp.LpVariable('Y')
prob +=Y , 'objective function'
prob += pp.lpSum([y[j,0] for j in range(Y_max)]) == Y, ""
for i in range(len(order)):
prob += pp.lpSum([x[i,j] for j in range(Y_max)]) == 1,'' #product i can only be placed in 1 bin
for j in range(Y_max):
prob += pp.lpSum([x[i,j]*w[i] for i in range(len(order))]) <= W*y[j,0],"" #weight constraint
for j in range(Y_max):
prob += pp.lpSum([x[i,j]*v[i] for i in range(len(order))]) <= V*y[j,0],"" #volume constraint
for j in range(Y_max):
for i in range(len(order)):
prob += x[i,j] <= y[j,0],"" #products i may not be placed in bin j if bin j is unnused.
prob += pp.lpSum([y[j,0] for i in range(len(order))]) >=Y_min,""
pp.LpSolverDefault.msg = 1
prob.solve()`
Second optimization: minimize the number of zones that the bin travels to (how to solve in linear optimization?)
Each product comes from 1 out of two zones (Zone 1 or Zone 2). We have a list of these zones z_i (e.g. Zone 1 <==> 1, Zone 2 <==> 0).
Under the constraint that the number of bins does not exceed the minimum number of bins (determined in first optimization), can I split the products over the bins such that the number of zones that each bin travels to is minimized?
Volume and weight constraints of first optimization still apply.
Ideally a bin would never travel to two zones, but in some cases it is forced to do so. (e.g. first optimization leads to 1 bin containing products of zone 1 and zone 2).
python algorithm optimization linear-programming bin-packing
add a comment |
I have a list of products (i), each with given weight and volume. The optimization goes in two steps, of which I have been unable to solve the second step.
First optimization: minimize number of bins used (solved)
Minimize number of bins used to pack list of products. I have fixed bins with with maximum volume and weight constraints. Python code:
prob = pp.LpProblem("algorithm",pp.LpMinimize) #pp=pulp solver
Y_max=10 #bins will not exceed this number
#Y_min = minimum number of bins (calculated)
# j = index of jth bin
# i = index of ith product
w=weights #list of weights w_i for product i
v=volumes #list of volumes v_i for product i
W=W_max #maximum weight of a bin
V=V_max #maximum volume of a bin
#len(order) = number of products
x=pp.LpVariable.dicts("x_i,j", ((i, j) for i in range(len(order)) for j in range(Y_max)),0,1,pp.LpBinary) #variable indicating if product i is placed in bin j
y=pp.LpVariable.dicts("y_j", ((j,0) for j in range(Y_max)),0,1,pp.LpBinary) #variable indicating if bin j is used or unused
Y=pp.LpVariable('Y')
prob +=Y , 'objective function'
prob += pp.lpSum([y[j,0] for j in range(Y_max)]) == Y, ""
for i in range(len(order)):
prob += pp.lpSum([x[i,j] for j in range(Y_max)]) == 1,'' #product i can only be placed in 1 bin
for j in range(Y_max):
prob += pp.lpSum([x[i,j]*w[i] for i in range(len(order))]) <= W*y[j,0],"" #weight constraint
for j in range(Y_max):
prob += pp.lpSum([x[i,j]*v[i] for i in range(len(order))]) <= V*y[j,0],"" #volume constraint
for j in range(Y_max):
for i in range(len(order)):
prob += x[i,j] <= y[j,0],"" #products i may not be placed in bin j if bin j is unnused.
prob += pp.lpSum([y[j,0] for i in range(len(order))]) >=Y_min,""
pp.LpSolverDefault.msg = 1
prob.solve()`
Second optimization: minimize the number of zones that the bin travels to (how to solve in linear optimization?)
Each product comes from 1 out of two zones (Zone 1 or Zone 2). We have a list of these zones z_i (e.g. Zone 1 <==> 1, Zone 2 <==> 0).
Under the constraint that the number of bins does not exceed the minimum number of bins (determined in first optimization), can I split the products over the bins such that the number of zones that each bin travels to is minimized?
Volume and weight constraints of first optimization still apply.
Ideally a bin would never travel to two zones, but in some cases it is forced to do so. (e.g. first optimization leads to 1 bin containing products of zone 1 and zone 2).
python algorithm optimization linear-programming bin-packing
1
if you model with variables how many bins used have products from 2 zones, you can then optimize the function sum([y[j,0] for j in range(Y_max)]) * Y_max + bins from 2 zones
– juvian
Nov 21 '18 at 15:32
add a comment |
I have a list of products (i), each with given weight and volume. The optimization goes in two steps, of which I have been unable to solve the second step.
First optimization: minimize number of bins used (solved)
Minimize number of bins used to pack list of products. I have fixed bins with with maximum volume and weight constraints. Python code:
prob = pp.LpProblem("algorithm",pp.LpMinimize) #pp=pulp solver
Y_max=10 #bins will not exceed this number
#Y_min = minimum number of bins (calculated)
# j = index of jth bin
# i = index of ith product
w=weights #list of weights w_i for product i
v=volumes #list of volumes v_i for product i
W=W_max #maximum weight of a bin
V=V_max #maximum volume of a bin
#len(order) = number of products
x=pp.LpVariable.dicts("x_i,j", ((i, j) for i in range(len(order)) for j in range(Y_max)),0,1,pp.LpBinary) #variable indicating if product i is placed in bin j
y=pp.LpVariable.dicts("y_j", ((j,0) for j in range(Y_max)),0,1,pp.LpBinary) #variable indicating if bin j is used or unused
Y=pp.LpVariable('Y')
prob +=Y , 'objective function'
prob += pp.lpSum([y[j,0] for j in range(Y_max)]) == Y, ""
for i in range(len(order)):
prob += pp.lpSum([x[i,j] for j in range(Y_max)]) == 1,'' #product i can only be placed in 1 bin
for j in range(Y_max):
prob += pp.lpSum([x[i,j]*w[i] for i in range(len(order))]) <= W*y[j,0],"" #weight constraint
for j in range(Y_max):
prob += pp.lpSum([x[i,j]*v[i] for i in range(len(order))]) <= V*y[j,0],"" #volume constraint
for j in range(Y_max):
for i in range(len(order)):
prob += x[i,j] <= y[j,0],"" #products i may not be placed in bin j if bin j is unnused.
prob += pp.lpSum([y[j,0] for i in range(len(order))]) >=Y_min,""
pp.LpSolverDefault.msg = 1
prob.solve()`
Second optimization: minimize the number of zones that the bin travels to (how to solve in linear optimization?)
Each product comes from 1 out of two zones (Zone 1 or Zone 2). We have a list of these zones z_i (e.g. Zone 1 <==> 1, Zone 2 <==> 0).
Under the constraint that the number of bins does not exceed the minimum number of bins (determined in first optimization), can I split the products over the bins such that the number of zones that each bin travels to is minimized?
Volume and weight constraints of first optimization still apply.
Ideally a bin would never travel to two zones, but in some cases it is forced to do so. (e.g. first optimization leads to 1 bin containing products of zone 1 and zone 2).
python algorithm optimization linear-programming bin-packing
I have a list of products (i), each with given weight and volume. The optimization goes in two steps, of which I have been unable to solve the second step.
First optimization: minimize number of bins used (solved)
Minimize number of bins used to pack list of products. I have fixed bins with with maximum volume and weight constraints. Python code:
prob = pp.LpProblem("algorithm",pp.LpMinimize) #pp=pulp solver
Y_max=10 #bins will not exceed this number
#Y_min = minimum number of bins (calculated)
# j = index of jth bin
# i = index of ith product
w=weights #list of weights w_i for product i
v=volumes #list of volumes v_i for product i
W=W_max #maximum weight of a bin
V=V_max #maximum volume of a bin
#len(order) = number of products
x=pp.LpVariable.dicts("x_i,j", ((i, j) for i in range(len(order)) for j in range(Y_max)),0,1,pp.LpBinary) #variable indicating if product i is placed in bin j
y=pp.LpVariable.dicts("y_j", ((j,0) for j in range(Y_max)),0,1,pp.LpBinary) #variable indicating if bin j is used or unused
Y=pp.LpVariable('Y')
prob +=Y , 'objective function'
prob += pp.lpSum([y[j,0] for j in range(Y_max)]) == Y, ""
for i in range(len(order)):
prob += pp.lpSum([x[i,j] for j in range(Y_max)]) == 1,'' #product i can only be placed in 1 bin
for j in range(Y_max):
prob += pp.lpSum([x[i,j]*w[i] for i in range(len(order))]) <= W*y[j,0],"" #weight constraint
for j in range(Y_max):
prob += pp.lpSum([x[i,j]*v[i] for i in range(len(order))]) <= V*y[j,0],"" #volume constraint
for j in range(Y_max):
for i in range(len(order)):
prob += x[i,j] <= y[j,0],"" #products i may not be placed in bin j if bin j is unnused.
prob += pp.lpSum([y[j,0] for i in range(len(order))]) >=Y_min,""
pp.LpSolverDefault.msg = 1
prob.solve()`
Second optimization: minimize the number of zones that the bin travels to (how to solve in linear optimization?)
Each product comes from 1 out of two zones (Zone 1 or Zone 2). We have a list of these zones z_i (e.g. Zone 1 <==> 1, Zone 2 <==> 0).
Under the constraint that the number of bins does not exceed the minimum number of bins (determined in first optimization), can I split the products over the bins such that the number of zones that each bin travels to is minimized?
Volume and weight constraints of first optimization still apply.
Ideally a bin would never travel to two zones, but in some cases it is forced to do so. (e.g. first optimization leads to 1 bin containing products of zone 1 and zone 2).
python algorithm optimization linear-programming bin-packing
python algorithm optimization linear-programming bin-packing
asked Nov 21 '18 at 12:39
Jobbe van der MaesenJobbe van der Maesen
182
182
1
if you model with variables how many bins used have products from 2 zones, you can then optimize the function sum([y[j,0] for j in range(Y_max)]) * Y_max + bins from 2 zones
– juvian
Nov 21 '18 at 15:32
add a comment |
1
if you model with variables how many bins used have products from 2 zones, you can then optimize the function sum([y[j,0] for j in range(Y_max)]) * Y_max + bins from 2 zones
– juvian
Nov 21 '18 at 15:32
1
1
if you model with variables how many bins used have products from 2 zones, you can then optimize the function sum([y[j,0] for j in range(Y_max)]) * Y_max + bins from 2 zones
– juvian
Nov 21 '18 at 15:32
if you model with variables how many bins used have products from 2 zones, you can then optimize the function sum([y[j,0] for j in range(Y_max)]) * Y_max + bins from 2 zones
– juvian
Nov 21 '18 at 15:32
add a comment |
1 Answer
1
active
oldest
votes
Below is one way of doing it - not necessarily the neatest or most efficient. Similar to that suggested by @juvian
If you slowly reduce the volume per bin, you'll find whilst it large you can fit in a small number of bins, with each bin only visiting a single zone. As bins get smaller you're forced to have bins travel to more than one zone, and as they get smaller again you're forced to use more bins.
Notice in the objective function:
prob += Y + pp.lpSum([two_zones[j] for j in range(Y_max)])/(Y_max+1)
We divide our secondary objective (No. of bins which have products from both zones) by the maximum No. of bins + 1. This way we always prioritise the primary objective of number of bins - even if every bin has items from different zones, the second sum can be at most Y_max
, so if we divide it by Y_max + 1
we get a value less than 1.0, so would prefer to reduct the number of used bins by 1. This is a common technique when you want to prioritize objectives.
import numpy as np
import pulp as pp
prob = pp.LpProblem("algorithm",pp.LpMinimize) #pp=pulp solver
Y_max = 5 #bins will not exceed this number
#Y_min = minimum number of bins (calculated)
# j = index of jth bin
# i = index of ith product
# Some dummy data
n_prod = 10
np.random.seed(0)
w = np.random.uniform(2.5, 10, n_prod) # product weights
v = np.random.uniform(0.1, 1, n_prod) # product volumes
W = 25 #maximum weight of a bin
V = 1.5 #maximum volume of a bin
z = np.random.randint(0, 2, n_prod) # product zones
x=pp.LpVariable.dicts("x_i,j", ((i, j) for i in range(n_prod) for j in range(Y_max)), cat='Binary') #variable indicating if product i is placed in bin j
y=pp.LpVariable.dicts("y_j", range(Y_max), cat='Binary') #variable indicating if bin j is used or unused
Y=pp.LpVariable('Y') # No. bins used
two_zones = pp.LpVariable.dicts("two_zones,j", range(Y_max), cat='Binary')
has_zone_0 = pp.LpVariable.dicts("has_zone_0,j", range(Y_max), cat='Binary')
has_zone_1 = pp.LpVariable.dicts("has_zone_1,j", range(Y_max), cat='Binary')
# Primary objective: minm No. bins, Secondary minimize bins that visit two zones
prob += Y + pp.lpSum([two_zones[j] for j in range(Y_max)])/(Y_max+1), 'objective function'
prob += pp.lpSum([y[j] for j in range(Y_max)]) == Y, 'set Y to No. bins'
for i in range(n_prod):
prob += pp.lpSum([x[i,j] for j in range(Y_max)]) == 1,'each product in 1 bin %s' % i
for j in range(Y_max):
prob += pp.lpSum([x[i,j]*w[i] for i in range(n_prod)]) <= W*y[j], 'weight constraint %s' % j
prob += pp.lpSum([x[i,j]*v[i] for i in range(n_prod)]) <= V*y[j], 'volume constraint %s' % j
for i in range(n_prod):
prob += x[i,j] <= y[j], 'products only placed in used bin %s_%s' % (j, i)
prob += has_zone_0[j] >= x[i,j]*(z[i] == 0), 'set has_zone_0 flag %s_%s' % (j, i)
prob += has_zone_1[j] >= x[i,j]*(z[i] == 1), 'set has_zone_1 flag %s_%s' % (j, i)
prob += two_zones[j] >= has_zone_0[j] + has_zone_1[j] - 1, 'set two_zones flag %s' % j
prob.solve()
has_zone_0_soln = np.array([has_zone_0[j].varValue for j in range(Y_max)])
has_zone_1_soln = np.array([has_zone_1[j].varValue for j in range(Y_max)])
two_zones_soln = np.array([two_zones[j].varValue for j in range(Y_max)])
y_soln = np.array([y[j].varValue for j in range(Y_max)])
# Print some output:
print("Status:" + str(pp.LpStatus[prob.status]))
print('z: ' + str(z))
print('Y: ' + str(Y.varValue))
print('y_soln: ' + str(y_soln))
print('Objective: ' + str(pp.value(prob.objective)))
print('has_zone_0_soln: ' + str(has_zone_0_soln))
print('has_zone_1_soln: ' + str(has_zone_1_soln))
print('two_zones_soln: ' + str(two_zones_soln))
add a comment |
Your Answer
StackExchange.ifUsing("editor", function () {
StackExchange.using("externalEditor", function () {
StackExchange.using("snippets", function () {
StackExchange.snippets.init();
});
});
}, "code-snippets");
StackExchange.ready(function() {
var channelOptions = {
tags: "".split(" "),
id: "1"
};
initTagRenderer("".split(" "), "".split(" "), channelOptions);
StackExchange.using("externalEditor", function() {
// Have to fire editor after snippets, if snippets enabled
if (StackExchange.settings.snippets.snippetsEnabled) {
StackExchange.using("snippets", function() {
createEditor();
});
}
else {
createEditor();
}
});
function createEditor() {
StackExchange.prepareEditor({
heartbeatType: 'answer',
autoActivateHeartbeat: false,
convertImagesToLinks: true,
noModals: true,
showLowRepImageUploadWarning: true,
reputationToPostImages: 10,
bindNavPrevention: true,
postfix: "",
imageUploader: {
brandingHtml: "Powered by u003ca class="icon-imgur-white" href="https://imgur.com/"u003eu003c/au003e",
contentPolicyHtml: "User contributions licensed under u003ca href="https://creativecommons.org/licenses/by-sa/3.0/"u003ecc by-sa 3.0 with attribution requiredu003c/au003e u003ca href="https://stackoverflow.com/legal/content-policy"u003e(content policy)u003c/au003e",
allowUrls: true
},
onDemand: true,
discardSelector: ".discard-answer"
,immediatelyShowMarkdownHelp:true
});
}
});
Sign up or log in
StackExchange.ready(function () {
StackExchange.helpers.onClickDraftSave('#login-link');
});
Sign up using Google
Sign up using Facebook
Sign up using Email and Password
Post as a guest
Required, but never shown
StackExchange.ready(
function () {
StackExchange.openid.initPostLogin('.new-post-login', 'https%3a%2f%2fstackoverflow.com%2fquestions%2f53412232%2fbin-packing-with-grouping%23new-answer', 'question_page');
}
);
Post as a guest
Required, but never shown
1 Answer
1
active
oldest
votes
1 Answer
1
active
oldest
votes
active
oldest
votes
active
oldest
votes
Below is one way of doing it - not necessarily the neatest or most efficient. Similar to that suggested by @juvian
If you slowly reduce the volume per bin, you'll find whilst it large you can fit in a small number of bins, with each bin only visiting a single zone. As bins get smaller you're forced to have bins travel to more than one zone, and as they get smaller again you're forced to use more bins.
Notice in the objective function:
prob += Y + pp.lpSum([two_zones[j] for j in range(Y_max)])/(Y_max+1)
We divide our secondary objective (No. of bins which have products from both zones) by the maximum No. of bins + 1. This way we always prioritise the primary objective of number of bins - even if every bin has items from different zones, the second sum can be at most Y_max
, so if we divide it by Y_max + 1
we get a value less than 1.0, so would prefer to reduct the number of used bins by 1. This is a common technique when you want to prioritize objectives.
import numpy as np
import pulp as pp
prob = pp.LpProblem("algorithm",pp.LpMinimize) #pp=pulp solver
Y_max = 5 #bins will not exceed this number
#Y_min = minimum number of bins (calculated)
# j = index of jth bin
# i = index of ith product
# Some dummy data
n_prod = 10
np.random.seed(0)
w = np.random.uniform(2.5, 10, n_prod) # product weights
v = np.random.uniform(0.1, 1, n_prod) # product volumes
W = 25 #maximum weight of a bin
V = 1.5 #maximum volume of a bin
z = np.random.randint(0, 2, n_prod) # product zones
x=pp.LpVariable.dicts("x_i,j", ((i, j) for i in range(n_prod) for j in range(Y_max)), cat='Binary') #variable indicating if product i is placed in bin j
y=pp.LpVariable.dicts("y_j", range(Y_max), cat='Binary') #variable indicating if bin j is used or unused
Y=pp.LpVariable('Y') # No. bins used
two_zones = pp.LpVariable.dicts("two_zones,j", range(Y_max), cat='Binary')
has_zone_0 = pp.LpVariable.dicts("has_zone_0,j", range(Y_max), cat='Binary')
has_zone_1 = pp.LpVariable.dicts("has_zone_1,j", range(Y_max), cat='Binary')
# Primary objective: minm No. bins, Secondary minimize bins that visit two zones
prob += Y + pp.lpSum([two_zones[j] for j in range(Y_max)])/(Y_max+1), 'objective function'
prob += pp.lpSum([y[j] for j in range(Y_max)]) == Y, 'set Y to No. bins'
for i in range(n_prod):
prob += pp.lpSum([x[i,j] for j in range(Y_max)]) == 1,'each product in 1 bin %s' % i
for j in range(Y_max):
prob += pp.lpSum([x[i,j]*w[i] for i in range(n_prod)]) <= W*y[j], 'weight constraint %s' % j
prob += pp.lpSum([x[i,j]*v[i] for i in range(n_prod)]) <= V*y[j], 'volume constraint %s' % j
for i in range(n_prod):
prob += x[i,j] <= y[j], 'products only placed in used bin %s_%s' % (j, i)
prob += has_zone_0[j] >= x[i,j]*(z[i] == 0), 'set has_zone_0 flag %s_%s' % (j, i)
prob += has_zone_1[j] >= x[i,j]*(z[i] == 1), 'set has_zone_1 flag %s_%s' % (j, i)
prob += two_zones[j] >= has_zone_0[j] + has_zone_1[j] - 1, 'set two_zones flag %s' % j
prob.solve()
has_zone_0_soln = np.array([has_zone_0[j].varValue for j in range(Y_max)])
has_zone_1_soln = np.array([has_zone_1[j].varValue for j in range(Y_max)])
two_zones_soln = np.array([two_zones[j].varValue for j in range(Y_max)])
y_soln = np.array([y[j].varValue for j in range(Y_max)])
# Print some output:
print("Status:" + str(pp.LpStatus[prob.status]))
print('z: ' + str(z))
print('Y: ' + str(Y.varValue))
print('y_soln: ' + str(y_soln))
print('Objective: ' + str(pp.value(prob.objective)))
print('has_zone_0_soln: ' + str(has_zone_0_soln))
print('has_zone_1_soln: ' + str(has_zone_1_soln))
print('two_zones_soln: ' + str(two_zones_soln))
add a comment |
Below is one way of doing it - not necessarily the neatest or most efficient. Similar to that suggested by @juvian
If you slowly reduce the volume per bin, you'll find whilst it large you can fit in a small number of bins, with each bin only visiting a single zone. As bins get smaller you're forced to have bins travel to more than one zone, and as they get smaller again you're forced to use more bins.
Notice in the objective function:
prob += Y + pp.lpSum([two_zones[j] for j in range(Y_max)])/(Y_max+1)
We divide our secondary objective (No. of bins which have products from both zones) by the maximum No. of bins + 1. This way we always prioritise the primary objective of number of bins - even if every bin has items from different zones, the second sum can be at most Y_max
, so if we divide it by Y_max + 1
we get a value less than 1.0, so would prefer to reduct the number of used bins by 1. This is a common technique when you want to prioritize objectives.
import numpy as np
import pulp as pp
prob = pp.LpProblem("algorithm",pp.LpMinimize) #pp=pulp solver
Y_max = 5 #bins will not exceed this number
#Y_min = minimum number of bins (calculated)
# j = index of jth bin
# i = index of ith product
# Some dummy data
n_prod = 10
np.random.seed(0)
w = np.random.uniform(2.5, 10, n_prod) # product weights
v = np.random.uniform(0.1, 1, n_prod) # product volumes
W = 25 #maximum weight of a bin
V = 1.5 #maximum volume of a bin
z = np.random.randint(0, 2, n_prod) # product zones
x=pp.LpVariable.dicts("x_i,j", ((i, j) for i in range(n_prod) for j in range(Y_max)), cat='Binary') #variable indicating if product i is placed in bin j
y=pp.LpVariable.dicts("y_j", range(Y_max), cat='Binary') #variable indicating if bin j is used or unused
Y=pp.LpVariable('Y') # No. bins used
two_zones = pp.LpVariable.dicts("two_zones,j", range(Y_max), cat='Binary')
has_zone_0 = pp.LpVariable.dicts("has_zone_0,j", range(Y_max), cat='Binary')
has_zone_1 = pp.LpVariable.dicts("has_zone_1,j", range(Y_max), cat='Binary')
# Primary objective: minm No. bins, Secondary minimize bins that visit two zones
prob += Y + pp.lpSum([two_zones[j] for j in range(Y_max)])/(Y_max+1), 'objective function'
prob += pp.lpSum([y[j] for j in range(Y_max)]) == Y, 'set Y to No. bins'
for i in range(n_prod):
prob += pp.lpSum([x[i,j] for j in range(Y_max)]) == 1,'each product in 1 bin %s' % i
for j in range(Y_max):
prob += pp.lpSum([x[i,j]*w[i] for i in range(n_prod)]) <= W*y[j], 'weight constraint %s' % j
prob += pp.lpSum([x[i,j]*v[i] for i in range(n_prod)]) <= V*y[j], 'volume constraint %s' % j
for i in range(n_prod):
prob += x[i,j] <= y[j], 'products only placed in used bin %s_%s' % (j, i)
prob += has_zone_0[j] >= x[i,j]*(z[i] == 0), 'set has_zone_0 flag %s_%s' % (j, i)
prob += has_zone_1[j] >= x[i,j]*(z[i] == 1), 'set has_zone_1 flag %s_%s' % (j, i)
prob += two_zones[j] >= has_zone_0[j] + has_zone_1[j] - 1, 'set two_zones flag %s' % j
prob.solve()
has_zone_0_soln = np.array([has_zone_0[j].varValue for j in range(Y_max)])
has_zone_1_soln = np.array([has_zone_1[j].varValue for j in range(Y_max)])
two_zones_soln = np.array([two_zones[j].varValue for j in range(Y_max)])
y_soln = np.array([y[j].varValue for j in range(Y_max)])
# Print some output:
print("Status:" + str(pp.LpStatus[prob.status]))
print('z: ' + str(z))
print('Y: ' + str(Y.varValue))
print('y_soln: ' + str(y_soln))
print('Objective: ' + str(pp.value(prob.objective)))
print('has_zone_0_soln: ' + str(has_zone_0_soln))
print('has_zone_1_soln: ' + str(has_zone_1_soln))
print('two_zones_soln: ' + str(two_zones_soln))
add a comment |
Below is one way of doing it - not necessarily the neatest or most efficient. Similar to that suggested by @juvian
If you slowly reduce the volume per bin, you'll find whilst it large you can fit in a small number of bins, with each bin only visiting a single zone. As bins get smaller you're forced to have bins travel to more than one zone, and as they get smaller again you're forced to use more bins.
Notice in the objective function:
prob += Y + pp.lpSum([two_zones[j] for j in range(Y_max)])/(Y_max+1)
We divide our secondary objective (No. of bins which have products from both zones) by the maximum No. of bins + 1. This way we always prioritise the primary objective of number of bins - even if every bin has items from different zones, the second sum can be at most Y_max
, so if we divide it by Y_max + 1
we get a value less than 1.0, so would prefer to reduct the number of used bins by 1. This is a common technique when you want to prioritize objectives.
import numpy as np
import pulp as pp
prob = pp.LpProblem("algorithm",pp.LpMinimize) #pp=pulp solver
Y_max = 5 #bins will not exceed this number
#Y_min = minimum number of bins (calculated)
# j = index of jth bin
# i = index of ith product
# Some dummy data
n_prod = 10
np.random.seed(0)
w = np.random.uniform(2.5, 10, n_prod) # product weights
v = np.random.uniform(0.1, 1, n_prod) # product volumes
W = 25 #maximum weight of a bin
V = 1.5 #maximum volume of a bin
z = np.random.randint(0, 2, n_prod) # product zones
x=pp.LpVariable.dicts("x_i,j", ((i, j) for i in range(n_prod) for j in range(Y_max)), cat='Binary') #variable indicating if product i is placed in bin j
y=pp.LpVariable.dicts("y_j", range(Y_max), cat='Binary') #variable indicating if bin j is used or unused
Y=pp.LpVariable('Y') # No. bins used
two_zones = pp.LpVariable.dicts("two_zones,j", range(Y_max), cat='Binary')
has_zone_0 = pp.LpVariable.dicts("has_zone_0,j", range(Y_max), cat='Binary')
has_zone_1 = pp.LpVariable.dicts("has_zone_1,j", range(Y_max), cat='Binary')
# Primary objective: minm No. bins, Secondary minimize bins that visit two zones
prob += Y + pp.lpSum([two_zones[j] for j in range(Y_max)])/(Y_max+1), 'objective function'
prob += pp.lpSum([y[j] for j in range(Y_max)]) == Y, 'set Y to No. bins'
for i in range(n_prod):
prob += pp.lpSum([x[i,j] for j in range(Y_max)]) == 1,'each product in 1 bin %s' % i
for j in range(Y_max):
prob += pp.lpSum([x[i,j]*w[i] for i in range(n_prod)]) <= W*y[j], 'weight constraint %s' % j
prob += pp.lpSum([x[i,j]*v[i] for i in range(n_prod)]) <= V*y[j], 'volume constraint %s' % j
for i in range(n_prod):
prob += x[i,j] <= y[j], 'products only placed in used bin %s_%s' % (j, i)
prob += has_zone_0[j] >= x[i,j]*(z[i] == 0), 'set has_zone_0 flag %s_%s' % (j, i)
prob += has_zone_1[j] >= x[i,j]*(z[i] == 1), 'set has_zone_1 flag %s_%s' % (j, i)
prob += two_zones[j] >= has_zone_0[j] + has_zone_1[j] - 1, 'set two_zones flag %s' % j
prob.solve()
has_zone_0_soln = np.array([has_zone_0[j].varValue for j in range(Y_max)])
has_zone_1_soln = np.array([has_zone_1[j].varValue for j in range(Y_max)])
two_zones_soln = np.array([two_zones[j].varValue for j in range(Y_max)])
y_soln = np.array([y[j].varValue for j in range(Y_max)])
# Print some output:
print("Status:" + str(pp.LpStatus[prob.status]))
print('z: ' + str(z))
print('Y: ' + str(Y.varValue))
print('y_soln: ' + str(y_soln))
print('Objective: ' + str(pp.value(prob.objective)))
print('has_zone_0_soln: ' + str(has_zone_0_soln))
print('has_zone_1_soln: ' + str(has_zone_1_soln))
print('two_zones_soln: ' + str(two_zones_soln))
Below is one way of doing it - not necessarily the neatest or most efficient. Similar to that suggested by @juvian
If you slowly reduce the volume per bin, you'll find whilst it large you can fit in a small number of bins, with each bin only visiting a single zone. As bins get smaller you're forced to have bins travel to more than one zone, and as they get smaller again you're forced to use more bins.
Notice in the objective function:
prob += Y + pp.lpSum([two_zones[j] for j in range(Y_max)])/(Y_max+1)
We divide our secondary objective (No. of bins which have products from both zones) by the maximum No. of bins + 1. This way we always prioritise the primary objective of number of bins - even if every bin has items from different zones, the second sum can be at most Y_max
, so if we divide it by Y_max + 1
we get a value less than 1.0, so would prefer to reduct the number of used bins by 1. This is a common technique when you want to prioritize objectives.
import numpy as np
import pulp as pp
prob = pp.LpProblem("algorithm",pp.LpMinimize) #pp=pulp solver
Y_max = 5 #bins will not exceed this number
#Y_min = minimum number of bins (calculated)
# j = index of jth bin
# i = index of ith product
# Some dummy data
n_prod = 10
np.random.seed(0)
w = np.random.uniform(2.5, 10, n_prod) # product weights
v = np.random.uniform(0.1, 1, n_prod) # product volumes
W = 25 #maximum weight of a bin
V = 1.5 #maximum volume of a bin
z = np.random.randint(0, 2, n_prod) # product zones
x=pp.LpVariable.dicts("x_i,j", ((i, j) for i in range(n_prod) for j in range(Y_max)), cat='Binary') #variable indicating if product i is placed in bin j
y=pp.LpVariable.dicts("y_j", range(Y_max), cat='Binary') #variable indicating if bin j is used or unused
Y=pp.LpVariable('Y') # No. bins used
two_zones = pp.LpVariable.dicts("two_zones,j", range(Y_max), cat='Binary')
has_zone_0 = pp.LpVariable.dicts("has_zone_0,j", range(Y_max), cat='Binary')
has_zone_1 = pp.LpVariable.dicts("has_zone_1,j", range(Y_max), cat='Binary')
# Primary objective: minm No. bins, Secondary minimize bins that visit two zones
prob += Y + pp.lpSum([two_zones[j] for j in range(Y_max)])/(Y_max+1), 'objective function'
prob += pp.lpSum([y[j] for j in range(Y_max)]) == Y, 'set Y to No. bins'
for i in range(n_prod):
prob += pp.lpSum([x[i,j] for j in range(Y_max)]) == 1,'each product in 1 bin %s' % i
for j in range(Y_max):
prob += pp.lpSum([x[i,j]*w[i] for i in range(n_prod)]) <= W*y[j], 'weight constraint %s' % j
prob += pp.lpSum([x[i,j]*v[i] for i in range(n_prod)]) <= V*y[j], 'volume constraint %s' % j
for i in range(n_prod):
prob += x[i,j] <= y[j], 'products only placed in used bin %s_%s' % (j, i)
prob += has_zone_0[j] >= x[i,j]*(z[i] == 0), 'set has_zone_0 flag %s_%s' % (j, i)
prob += has_zone_1[j] >= x[i,j]*(z[i] == 1), 'set has_zone_1 flag %s_%s' % (j, i)
prob += two_zones[j] >= has_zone_0[j] + has_zone_1[j] - 1, 'set two_zones flag %s' % j
prob.solve()
has_zone_0_soln = np.array([has_zone_0[j].varValue for j in range(Y_max)])
has_zone_1_soln = np.array([has_zone_1[j].varValue for j in range(Y_max)])
two_zones_soln = np.array([two_zones[j].varValue for j in range(Y_max)])
y_soln = np.array([y[j].varValue for j in range(Y_max)])
# Print some output:
print("Status:" + str(pp.LpStatus[prob.status]))
print('z: ' + str(z))
print('Y: ' + str(Y.varValue))
print('y_soln: ' + str(y_soln))
print('Objective: ' + str(pp.value(prob.objective)))
print('has_zone_0_soln: ' + str(has_zone_0_soln))
print('has_zone_1_soln: ' + str(has_zone_1_soln))
print('two_zones_soln: ' + str(two_zones_soln))
answered Nov 21 '18 at 22:15


kabdullakabdulla
2,2571725
2,2571725
add a comment |
add a comment |
Thanks for contributing an answer to Stack Overflow!
- Please be sure to answer the question. Provide details and share your research!
But avoid …
- Asking for help, clarification, or responding to other answers.
- Making statements based on opinion; back them up with references or personal experience.
To learn more, see our tips on writing great answers.
Sign up or log in
StackExchange.ready(function () {
StackExchange.helpers.onClickDraftSave('#login-link');
});
Sign up using Google
Sign up using Facebook
Sign up using Email and Password
Post as a guest
Required, but never shown
StackExchange.ready(
function () {
StackExchange.openid.initPostLogin('.new-post-login', 'https%3a%2f%2fstackoverflow.com%2fquestions%2f53412232%2fbin-packing-with-grouping%23new-answer', 'question_page');
}
);
Post as a guest
Required, but never shown
Sign up or log in
StackExchange.ready(function () {
StackExchange.helpers.onClickDraftSave('#login-link');
});
Sign up using Google
Sign up using Facebook
Sign up using Email and Password
Post as a guest
Required, but never shown
Sign up or log in
StackExchange.ready(function () {
StackExchange.helpers.onClickDraftSave('#login-link');
});
Sign up using Google
Sign up using Facebook
Sign up using Email and Password
Post as a guest
Required, but never shown
Sign up or log in
StackExchange.ready(function () {
StackExchange.helpers.onClickDraftSave('#login-link');
});
Sign up using Google
Sign up using Facebook
Sign up using Email and Password
Sign up using Google
Sign up using Facebook
Sign up using Email and Password
Post as a guest
Required, but never shown
Required, but never shown
Required, but never shown
Required, but never shown
Required, but never shown
Required, but never shown
Required, but never shown
Required, but never shown
Required, but never shown
G3 Y6qxs2,4IdNJjOoARdFIw2C8N7eo,J0RWWGy,KF3uQAkbE 2v6VC2CykYgazY,jk4,9t3H,5J3gC
1
if you model with variables how many bins used have products from 2 zones, you can then optimize the function sum([y[j,0] for j in range(Y_max)]) * Y_max + bins from 2 zones
– juvian
Nov 21 '18 at 15:32