EvalSpec input_fn : case where the feature is an array
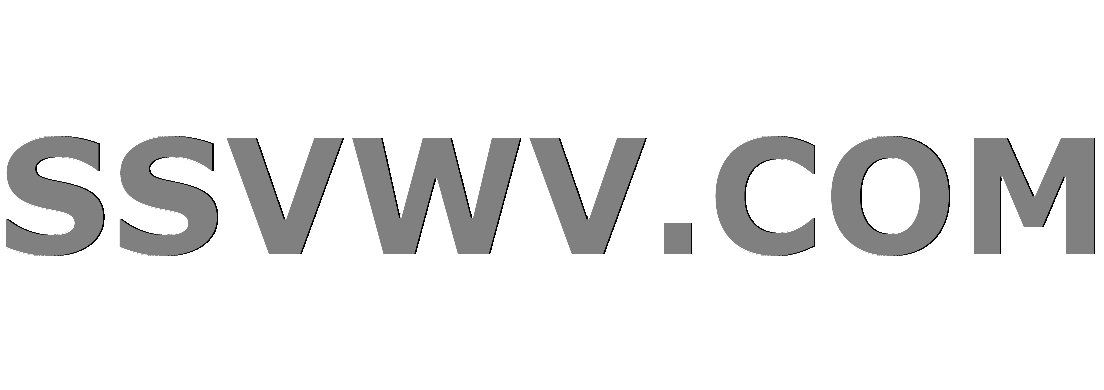
Multi tool use
I have some trouble understanding some details of the Estimator API and tf.estimator.EvalSpec
.
In an EvalSpec
, the user is supposed to give a input_fn
. A call to input_fn is supposed to return A tuple (features, labels)
.
As far as I understand, the features can be a dictionary keyed by "feature name" and whose values are a tensor of values. For instance, if I have a batch of 100 examples and a feature called "weight" I will create an entry in the feature dictionary that is a tensor of shape (100,1), with key weight, and with all the weights for all the examples, right?
However:
- what if my initial feature is already a tensor, like "size" which is a an array of 3 double values? How can I input it via the input_fn ?
And the question I'm mostly interested in:
- what if my initial feature is a variable-length array ? For instance my feature could be "prices of all purchased products", and it would be a variable-length array of doubles (this correspond to tf.io.VarLenFeature in feature specs). How can I send several examples of this via the input_fn ?
Are these types of features "compatible" with the Estimator API ?
thanks!
tensorflow tensorflow-datasets tensorflow-estimator
add a comment |
I have some trouble understanding some details of the Estimator API and tf.estimator.EvalSpec
.
In an EvalSpec
, the user is supposed to give a input_fn
. A call to input_fn is supposed to return A tuple (features, labels)
.
As far as I understand, the features can be a dictionary keyed by "feature name" and whose values are a tensor of values. For instance, if I have a batch of 100 examples and a feature called "weight" I will create an entry in the feature dictionary that is a tensor of shape (100,1), with key weight, and with all the weights for all the examples, right?
However:
- what if my initial feature is already a tensor, like "size" which is a an array of 3 double values? How can I input it via the input_fn ?
And the question I'm mostly interested in:
- what if my initial feature is a variable-length array ? For instance my feature could be "prices of all purchased products", and it would be a variable-length array of doubles (this correspond to tf.io.VarLenFeature in feature specs). How can I send several examples of this via the input_fn ?
Are these types of features "compatible" with the Estimator API ?
thanks!
tensorflow tensorflow-datasets tensorflow-estimator
add a comment |
I have some trouble understanding some details of the Estimator API and tf.estimator.EvalSpec
.
In an EvalSpec
, the user is supposed to give a input_fn
. A call to input_fn is supposed to return A tuple (features, labels)
.
As far as I understand, the features can be a dictionary keyed by "feature name" and whose values are a tensor of values. For instance, if I have a batch of 100 examples and a feature called "weight" I will create an entry in the feature dictionary that is a tensor of shape (100,1), with key weight, and with all the weights for all the examples, right?
However:
- what if my initial feature is already a tensor, like "size" which is a an array of 3 double values? How can I input it via the input_fn ?
And the question I'm mostly interested in:
- what if my initial feature is a variable-length array ? For instance my feature could be "prices of all purchased products", and it would be a variable-length array of doubles (this correspond to tf.io.VarLenFeature in feature specs). How can I send several examples of this via the input_fn ?
Are these types of features "compatible" with the Estimator API ?
thanks!
tensorflow tensorflow-datasets tensorflow-estimator
I have some trouble understanding some details of the Estimator API and tf.estimator.EvalSpec
.
In an EvalSpec
, the user is supposed to give a input_fn
. A call to input_fn is supposed to return A tuple (features, labels)
.
As far as I understand, the features can be a dictionary keyed by "feature name" and whose values are a tensor of values. For instance, if I have a batch of 100 examples and a feature called "weight" I will create an entry in the feature dictionary that is a tensor of shape (100,1), with key weight, and with all the weights for all the examples, right?
However:
- what if my initial feature is already a tensor, like "size" which is a an array of 3 double values? How can I input it via the input_fn ?
And the question I'm mostly interested in:
- what if my initial feature is a variable-length array ? For instance my feature could be "prices of all purchased products", and it would be a variable-length array of doubles (this correspond to tf.io.VarLenFeature in feature specs). How can I send several examples of this via the input_fn ?
Are these types of features "compatible" with the Estimator API ?
thanks!
tensorflow tensorflow-datasets tensorflow-estimator
tensorflow tensorflow-datasets tensorflow-estimator
asked Nov 20 '18 at 20:42


lezebulonlezebulon
3,28373063
3,28373063
add a comment |
add a comment |
1 Answer
1
active
oldest
votes
I am also new to the Estimator
API, but I have learned quite a lot with the S.O. community and will try to answer your question.
First, I would like to point you to this colab. This is currently the convention I use for my Estimator
s.
You are correct in that the input_fn
for both the TRAIN
and EVAL
modes are meant to be tuples in form (features, labels)
.
So let us tackle your first question:
what if my initial feature is already a tensor, like "size" which is a an array of 3 double values? How can I input it via the input_fn ?
Well this requires me to back track a bit, to your input of:
batch of 100 examples and a feature called "weight" I will create an entry in the feature dictionary that is a tensor of shape (100,1),
To make sure I understand correctly, you are saying that, what if instead of a Tensor
with shape [100, 1]
, you have a Tensor
or [100, <size>]
, in this case 3 doubles, so [100, 3]
?
Well if that is the case, that is no problem at all. In the linked colab a single example of the input has shape [20, 7]
. So a Tensor
of [3]
is straight forward.
The short answer is that whatever you specify as the features
part of the tuple is passed to model_fn
. So you want to pass a Tensor
of [batch_size, size]
you return a tuple of ([batch_size, size], labels)
. However, as another user pointed out to me on S.O. I will impart you with the same advice - use dictionaries e.g.
my_data = # Tensor with shape [batch_size, size]
features = {'my_data': my_data}
...
return (features, labels)
For reference, let us example the input_fn
of the colab, where I do the same things as advised above:
def input_fn(filenames:list, params):
mode = params['mode'] if 'mode' in params else 'train'
batch_size = params['batch_size']
shuffle(filenames) # <--- far more efficient than tf dataset shuffle
dataset = tf.data.TFRecordDataset(filenames)
# using fio's SCHEMA fill the TF Feature placeholders with values
dataset = dataset.map(lambda record: fio.from_record(record))
# using fio's SCHEMA restructure and unwrap (if possible) features (because tf records require wrapping everything into a list)
dataset = dataset.map(lambda context, features: fio.reconstitute((context, features)))
# dataset should be a tuple of (features, labels)
dataset = dataset.map(lambda context, features: (
{"input_tensors": features[I_FEATURE]}, # features <--- wrapping it in a dictionary
features[O_FEATURE] # labels
)
)
For simplicity, I will assume you are using tf.data.Dataset
. If your data is not stored as TF Record
s, you will need to replace line 1.
1. dataset = tf.data.TFRecordDataset(filenames)
2. dataset = dataset.map(lambda record: fio.from_record(record))
3. dataset = dataset.map(lambda context, features: fio.reconstitute((context, features)))
with however you construct your dataset, be it FeatureColumn
, from_tensor_slices
, etc and remove lines 2 and 3 since you do not need to recover your (Sequence)Example
from TF Record
s.
Now let us address your second question, variable length arrays.
It is just the same as above! wrap it in a dictionary and return it.
This is true with the notable exception of recovering your SequenceExample
from TF Record
s, where you will need VarLenFeature
add a comment |
Your Answer
StackExchange.ifUsing("editor", function () {
StackExchange.using("externalEditor", function () {
StackExchange.using("snippets", function () {
StackExchange.snippets.init();
});
});
}, "code-snippets");
StackExchange.ready(function() {
var channelOptions = {
tags: "".split(" "),
id: "1"
};
initTagRenderer("".split(" "), "".split(" "), channelOptions);
StackExchange.using("externalEditor", function() {
// Have to fire editor after snippets, if snippets enabled
if (StackExchange.settings.snippets.snippetsEnabled) {
StackExchange.using("snippets", function() {
createEditor();
});
}
else {
createEditor();
}
});
function createEditor() {
StackExchange.prepareEditor({
heartbeatType: 'answer',
autoActivateHeartbeat: false,
convertImagesToLinks: true,
noModals: true,
showLowRepImageUploadWarning: true,
reputationToPostImages: 10,
bindNavPrevention: true,
postfix: "",
imageUploader: {
brandingHtml: "Powered by u003ca class="icon-imgur-white" href="https://imgur.com/"u003eu003c/au003e",
contentPolicyHtml: "User contributions licensed under u003ca href="https://creativecommons.org/licenses/by-sa/3.0/"u003ecc by-sa 3.0 with attribution requiredu003c/au003e u003ca href="https://stackoverflow.com/legal/content-policy"u003e(content policy)u003c/au003e",
allowUrls: true
},
onDemand: true,
discardSelector: ".discard-answer"
,immediatelyShowMarkdownHelp:true
});
}
});
Sign up or log in
StackExchange.ready(function () {
StackExchange.helpers.onClickDraftSave('#login-link');
});
Sign up using Google
Sign up using Facebook
Sign up using Email and Password
Post as a guest
Required, but never shown
StackExchange.ready(
function () {
StackExchange.openid.initPostLogin('.new-post-login', 'https%3a%2f%2fstackoverflow.com%2fquestions%2f53401198%2fevalspec-input-fn-case-where-the-feature-is-an-array%23new-answer', 'question_page');
}
);
Post as a guest
Required, but never shown
1 Answer
1
active
oldest
votes
1 Answer
1
active
oldest
votes
active
oldest
votes
active
oldest
votes
I am also new to the Estimator
API, but I have learned quite a lot with the S.O. community and will try to answer your question.
First, I would like to point you to this colab. This is currently the convention I use for my Estimator
s.
You are correct in that the input_fn
for both the TRAIN
and EVAL
modes are meant to be tuples in form (features, labels)
.
So let us tackle your first question:
what if my initial feature is already a tensor, like "size" which is a an array of 3 double values? How can I input it via the input_fn ?
Well this requires me to back track a bit, to your input of:
batch of 100 examples and a feature called "weight" I will create an entry in the feature dictionary that is a tensor of shape (100,1),
To make sure I understand correctly, you are saying that, what if instead of a Tensor
with shape [100, 1]
, you have a Tensor
or [100, <size>]
, in this case 3 doubles, so [100, 3]
?
Well if that is the case, that is no problem at all. In the linked colab a single example of the input has shape [20, 7]
. So a Tensor
of [3]
is straight forward.
The short answer is that whatever you specify as the features
part of the tuple is passed to model_fn
. So you want to pass a Tensor
of [batch_size, size]
you return a tuple of ([batch_size, size], labels)
. However, as another user pointed out to me on S.O. I will impart you with the same advice - use dictionaries e.g.
my_data = # Tensor with shape [batch_size, size]
features = {'my_data': my_data}
...
return (features, labels)
For reference, let us example the input_fn
of the colab, where I do the same things as advised above:
def input_fn(filenames:list, params):
mode = params['mode'] if 'mode' in params else 'train'
batch_size = params['batch_size']
shuffle(filenames) # <--- far more efficient than tf dataset shuffle
dataset = tf.data.TFRecordDataset(filenames)
# using fio's SCHEMA fill the TF Feature placeholders with values
dataset = dataset.map(lambda record: fio.from_record(record))
# using fio's SCHEMA restructure and unwrap (if possible) features (because tf records require wrapping everything into a list)
dataset = dataset.map(lambda context, features: fio.reconstitute((context, features)))
# dataset should be a tuple of (features, labels)
dataset = dataset.map(lambda context, features: (
{"input_tensors": features[I_FEATURE]}, # features <--- wrapping it in a dictionary
features[O_FEATURE] # labels
)
)
For simplicity, I will assume you are using tf.data.Dataset
. If your data is not stored as TF Record
s, you will need to replace line 1.
1. dataset = tf.data.TFRecordDataset(filenames)
2. dataset = dataset.map(lambda record: fio.from_record(record))
3. dataset = dataset.map(lambda context, features: fio.reconstitute((context, features)))
with however you construct your dataset, be it FeatureColumn
, from_tensor_slices
, etc and remove lines 2 and 3 since you do not need to recover your (Sequence)Example
from TF Record
s.
Now let us address your second question, variable length arrays.
It is just the same as above! wrap it in a dictionary and return it.
This is true with the notable exception of recovering your SequenceExample
from TF Record
s, where you will need VarLenFeature
add a comment |
I am also new to the Estimator
API, but I have learned quite a lot with the S.O. community and will try to answer your question.
First, I would like to point you to this colab. This is currently the convention I use for my Estimator
s.
You are correct in that the input_fn
for both the TRAIN
and EVAL
modes are meant to be tuples in form (features, labels)
.
So let us tackle your first question:
what if my initial feature is already a tensor, like "size" which is a an array of 3 double values? How can I input it via the input_fn ?
Well this requires me to back track a bit, to your input of:
batch of 100 examples and a feature called "weight" I will create an entry in the feature dictionary that is a tensor of shape (100,1),
To make sure I understand correctly, you are saying that, what if instead of a Tensor
with shape [100, 1]
, you have a Tensor
or [100, <size>]
, in this case 3 doubles, so [100, 3]
?
Well if that is the case, that is no problem at all. In the linked colab a single example of the input has shape [20, 7]
. So a Tensor
of [3]
is straight forward.
The short answer is that whatever you specify as the features
part of the tuple is passed to model_fn
. So you want to pass a Tensor
of [batch_size, size]
you return a tuple of ([batch_size, size], labels)
. However, as another user pointed out to me on S.O. I will impart you with the same advice - use dictionaries e.g.
my_data = # Tensor with shape [batch_size, size]
features = {'my_data': my_data}
...
return (features, labels)
For reference, let us example the input_fn
of the colab, where I do the same things as advised above:
def input_fn(filenames:list, params):
mode = params['mode'] if 'mode' in params else 'train'
batch_size = params['batch_size']
shuffle(filenames) # <--- far more efficient than tf dataset shuffle
dataset = tf.data.TFRecordDataset(filenames)
# using fio's SCHEMA fill the TF Feature placeholders with values
dataset = dataset.map(lambda record: fio.from_record(record))
# using fio's SCHEMA restructure and unwrap (if possible) features (because tf records require wrapping everything into a list)
dataset = dataset.map(lambda context, features: fio.reconstitute((context, features)))
# dataset should be a tuple of (features, labels)
dataset = dataset.map(lambda context, features: (
{"input_tensors": features[I_FEATURE]}, # features <--- wrapping it in a dictionary
features[O_FEATURE] # labels
)
)
For simplicity, I will assume you are using tf.data.Dataset
. If your data is not stored as TF Record
s, you will need to replace line 1.
1. dataset = tf.data.TFRecordDataset(filenames)
2. dataset = dataset.map(lambda record: fio.from_record(record))
3. dataset = dataset.map(lambda context, features: fio.reconstitute((context, features)))
with however you construct your dataset, be it FeatureColumn
, from_tensor_slices
, etc and remove lines 2 and 3 since you do not need to recover your (Sequence)Example
from TF Record
s.
Now let us address your second question, variable length arrays.
It is just the same as above! wrap it in a dictionary and return it.
This is true with the notable exception of recovering your SequenceExample
from TF Record
s, where you will need VarLenFeature
add a comment |
I am also new to the Estimator
API, but I have learned quite a lot with the S.O. community and will try to answer your question.
First, I would like to point you to this colab. This is currently the convention I use for my Estimator
s.
You are correct in that the input_fn
for both the TRAIN
and EVAL
modes are meant to be tuples in form (features, labels)
.
So let us tackle your first question:
what if my initial feature is already a tensor, like "size" which is a an array of 3 double values? How can I input it via the input_fn ?
Well this requires me to back track a bit, to your input of:
batch of 100 examples and a feature called "weight" I will create an entry in the feature dictionary that is a tensor of shape (100,1),
To make sure I understand correctly, you are saying that, what if instead of a Tensor
with shape [100, 1]
, you have a Tensor
or [100, <size>]
, in this case 3 doubles, so [100, 3]
?
Well if that is the case, that is no problem at all. In the linked colab a single example of the input has shape [20, 7]
. So a Tensor
of [3]
is straight forward.
The short answer is that whatever you specify as the features
part of the tuple is passed to model_fn
. So you want to pass a Tensor
of [batch_size, size]
you return a tuple of ([batch_size, size], labels)
. However, as another user pointed out to me on S.O. I will impart you with the same advice - use dictionaries e.g.
my_data = # Tensor with shape [batch_size, size]
features = {'my_data': my_data}
...
return (features, labels)
For reference, let us example the input_fn
of the colab, where I do the same things as advised above:
def input_fn(filenames:list, params):
mode = params['mode'] if 'mode' in params else 'train'
batch_size = params['batch_size']
shuffle(filenames) # <--- far more efficient than tf dataset shuffle
dataset = tf.data.TFRecordDataset(filenames)
# using fio's SCHEMA fill the TF Feature placeholders with values
dataset = dataset.map(lambda record: fio.from_record(record))
# using fio's SCHEMA restructure and unwrap (if possible) features (because tf records require wrapping everything into a list)
dataset = dataset.map(lambda context, features: fio.reconstitute((context, features)))
# dataset should be a tuple of (features, labels)
dataset = dataset.map(lambda context, features: (
{"input_tensors": features[I_FEATURE]}, # features <--- wrapping it in a dictionary
features[O_FEATURE] # labels
)
)
For simplicity, I will assume you are using tf.data.Dataset
. If your data is not stored as TF Record
s, you will need to replace line 1.
1. dataset = tf.data.TFRecordDataset(filenames)
2. dataset = dataset.map(lambda record: fio.from_record(record))
3. dataset = dataset.map(lambda context, features: fio.reconstitute((context, features)))
with however you construct your dataset, be it FeatureColumn
, from_tensor_slices
, etc and remove lines 2 and 3 since you do not need to recover your (Sequence)Example
from TF Record
s.
Now let us address your second question, variable length arrays.
It is just the same as above! wrap it in a dictionary and return it.
This is true with the notable exception of recovering your SequenceExample
from TF Record
s, where you will need VarLenFeature
I am also new to the Estimator
API, but I have learned quite a lot with the S.O. community and will try to answer your question.
First, I would like to point you to this colab. This is currently the convention I use for my Estimator
s.
You are correct in that the input_fn
for both the TRAIN
and EVAL
modes are meant to be tuples in form (features, labels)
.
So let us tackle your first question:
what if my initial feature is already a tensor, like "size" which is a an array of 3 double values? How can I input it via the input_fn ?
Well this requires me to back track a bit, to your input of:
batch of 100 examples and a feature called "weight" I will create an entry in the feature dictionary that is a tensor of shape (100,1),
To make sure I understand correctly, you are saying that, what if instead of a Tensor
with shape [100, 1]
, you have a Tensor
or [100, <size>]
, in this case 3 doubles, so [100, 3]
?
Well if that is the case, that is no problem at all. In the linked colab a single example of the input has shape [20, 7]
. So a Tensor
of [3]
is straight forward.
The short answer is that whatever you specify as the features
part of the tuple is passed to model_fn
. So you want to pass a Tensor
of [batch_size, size]
you return a tuple of ([batch_size, size], labels)
. However, as another user pointed out to me on S.O. I will impart you with the same advice - use dictionaries e.g.
my_data = # Tensor with shape [batch_size, size]
features = {'my_data': my_data}
...
return (features, labels)
For reference, let us example the input_fn
of the colab, where I do the same things as advised above:
def input_fn(filenames:list, params):
mode = params['mode'] if 'mode' in params else 'train'
batch_size = params['batch_size']
shuffle(filenames) # <--- far more efficient than tf dataset shuffle
dataset = tf.data.TFRecordDataset(filenames)
# using fio's SCHEMA fill the TF Feature placeholders with values
dataset = dataset.map(lambda record: fio.from_record(record))
# using fio's SCHEMA restructure and unwrap (if possible) features (because tf records require wrapping everything into a list)
dataset = dataset.map(lambda context, features: fio.reconstitute((context, features)))
# dataset should be a tuple of (features, labels)
dataset = dataset.map(lambda context, features: (
{"input_tensors": features[I_FEATURE]}, # features <--- wrapping it in a dictionary
features[O_FEATURE] # labels
)
)
For simplicity, I will assume you are using tf.data.Dataset
. If your data is not stored as TF Record
s, you will need to replace line 1.
1. dataset = tf.data.TFRecordDataset(filenames)
2. dataset = dataset.map(lambda record: fio.from_record(record))
3. dataset = dataset.map(lambda context, features: fio.reconstitute((context, features)))
with however you construct your dataset, be it FeatureColumn
, from_tensor_slices
, etc and remove lines 2 and 3 since you do not need to recover your (Sequence)Example
from TF Record
s.
Now let us address your second question, variable length arrays.
It is just the same as above! wrap it in a dictionary and return it.
This is true with the notable exception of recovering your SequenceExample
from TF Record
s, where you will need VarLenFeature
answered Nov 21 '18 at 14:59


SumNeuronSumNeuron
1,228826
1,228826
add a comment |
add a comment |
Thanks for contributing an answer to Stack Overflow!
- Please be sure to answer the question. Provide details and share your research!
But avoid …
- Asking for help, clarification, or responding to other answers.
- Making statements based on opinion; back them up with references or personal experience.
To learn more, see our tips on writing great answers.
Sign up or log in
StackExchange.ready(function () {
StackExchange.helpers.onClickDraftSave('#login-link');
});
Sign up using Google
Sign up using Facebook
Sign up using Email and Password
Post as a guest
Required, but never shown
StackExchange.ready(
function () {
StackExchange.openid.initPostLogin('.new-post-login', 'https%3a%2f%2fstackoverflow.com%2fquestions%2f53401198%2fevalspec-input-fn-case-where-the-feature-is-an-array%23new-answer', 'question_page');
}
);
Post as a guest
Required, but never shown
Sign up or log in
StackExchange.ready(function () {
StackExchange.helpers.onClickDraftSave('#login-link');
});
Sign up using Google
Sign up using Facebook
Sign up using Email and Password
Post as a guest
Required, but never shown
Sign up or log in
StackExchange.ready(function () {
StackExchange.helpers.onClickDraftSave('#login-link');
});
Sign up using Google
Sign up using Facebook
Sign up using Email and Password
Post as a guest
Required, but never shown
Sign up or log in
StackExchange.ready(function () {
StackExchange.helpers.onClickDraftSave('#login-link');
});
Sign up using Google
Sign up using Facebook
Sign up using Email and Password
Sign up using Google
Sign up using Facebook
Sign up using Email and Password
Post as a guest
Required, but never shown
Required, but never shown
Required, but never shown
Required, but never shown
Required, but never shown
Required, but never shown
Required, but never shown
Required, but never shown
Required, but never shown
9OAwnp4QPOnc9