fixed-size topics vector in gensim LDA topic modelling for finding similar texts
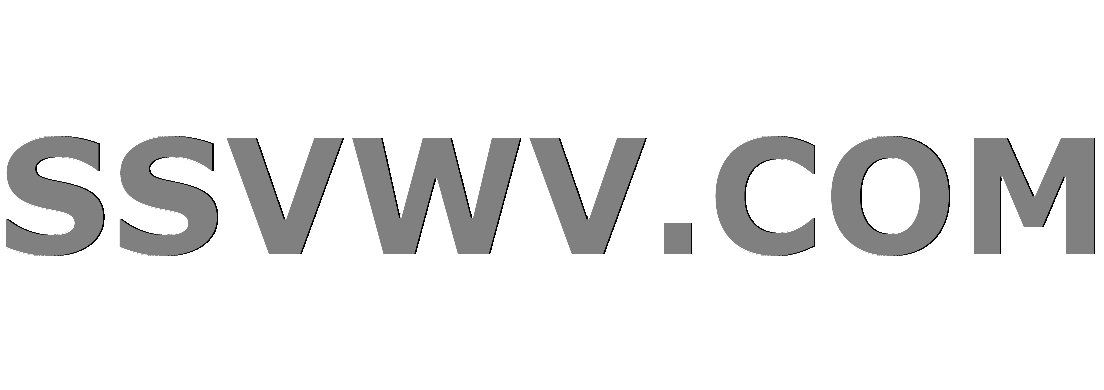
Multi tool use
I use gensim LDA topic modelling to find topics for each document and to check the similarity between documents by comparing the received topics vectors.
Each document is given a different number of matching topics, so the comparison of the vector (by cosine similarity) is incorrect because vectors of the same length are required.
This is the related code:
lda_model_bow = models.LdaModel(corpus=bow_corpus, id2word=dictionary, num_topics=3, passes=1, random_state=47)
#---------------Calculating and Viewing the topics----------------------------
vec_bows = [dictionary.doc2bow(filtered_text.split()) for filtered_text in filtered_texts]
vec_lda_topics=[lda_model_bow[vec_bow] for vec_bow in vec_bows]
for id,vec_lda_topic in enumerate(vec_lda_topics):
print ('document ' ,id, 'topics: ', vec_lda_topic)
The output vectors is:
document 0 topics: [(1, 0.25697246), (2, 0.08026043), (3, 0.65391296)]
document 1 topics: [(2, 0.93666667)]
document 2 topics: [(2, 0.07910537), (3, 0.20132676)]
.....
As you can see, each vector has a different length, so it is not possible to perform cosine similarity between them.
I would like the output to be:
document 0 topics: [(1, 0.25697246), (2, 0.08026043), (3, 0.65391296)]
document 1 topics: [(1, 0.0), (2, 0.93666667), (3, 0.0)]
document 2 topics: [(1, 0.0), (2, 0.07910537), (3, 0.20132676)]
.....
Any ideas how to do it? tnx
python gensim lda topic-modeling cosine-similarity
add a comment |
I use gensim LDA topic modelling to find topics for each document and to check the similarity between documents by comparing the received topics vectors.
Each document is given a different number of matching topics, so the comparison of the vector (by cosine similarity) is incorrect because vectors of the same length are required.
This is the related code:
lda_model_bow = models.LdaModel(corpus=bow_corpus, id2word=dictionary, num_topics=3, passes=1, random_state=47)
#---------------Calculating and Viewing the topics----------------------------
vec_bows = [dictionary.doc2bow(filtered_text.split()) for filtered_text in filtered_texts]
vec_lda_topics=[lda_model_bow[vec_bow] for vec_bow in vec_bows]
for id,vec_lda_topic in enumerate(vec_lda_topics):
print ('document ' ,id, 'topics: ', vec_lda_topic)
The output vectors is:
document 0 topics: [(1, 0.25697246), (2, 0.08026043), (3, 0.65391296)]
document 1 topics: [(2, 0.93666667)]
document 2 topics: [(2, 0.07910537), (3, 0.20132676)]
.....
As you can see, each vector has a different length, so it is not possible to perform cosine similarity between them.
I would like the output to be:
document 0 topics: [(1, 0.25697246), (2, 0.08026043), (3, 0.65391296)]
document 1 topics: [(1, 0.0), (2, 0.93666667), (3, 0.0)]
document 2 topics: [(1, 0.0), (2, 0.07910537), (3, 0.20132676)]
.....
Any ideas how to do it? tnx
python gensim lda topic-modeling cosine-similarity
add a comment |
I use gensim LDA topic modelling to find topics for each document and to check the similarity between documents by comparing the received topics vectors.
Each document is given a different number of matching topics, so the comparison of the vector (by cosine similarity) is incorrect because vectors of the same length are required.
This is the related code:
lda_model_bow = models.LdaModel(corpus=bow_corpus, id2word=dictionary, num_topics=3, passes=1, random_state=47)
#---------------Calculating and Viewing the topics----------------------------
vec_bows = [dictionary.doc2bow(filtered_text.split()) for filtered_text in filtered_texts]
vec_lda_topics=[lda_model_bow[vec_bow] for vec_bow in vec_bows]
for id,vec_lda_topic in enumerate(vec_lda_topics):
print ('document ' ,id, 'topics: ', vec_lda_topic)
The output vectors is:
document 0 topics: [(1, 0.25697246), (2, 0.08026043), (3, 0.65391296)]
document 1 topics: [(2, 0.93666667)]
document 2 topics: [(2, 0.07910537), (3, 0.20132676)]
.....
As you can see, each vector has a different length, so it is not possible to perform cosine similarity between them.
I would like the output to be:
document 0 topics: [(1, 0.25697246), (2, 0.08026043), (3, 0.65391296)]
document 1 topics: [(1, 0.0), (2, 0.93666667), (3, 0.0)]
document 2 topics: [(1, 0.0), (2, 0.07910537), (3, 0.20132676)]
.....
Any ideas how to do it? tnx
python gensim lda topic-modeling cosine-similarity
I use gensim LDA topic modelling to find topics for each document and to check the similarity between documents by comparing the received topics vectors.
Each document is given a different number of matching topics, so the comparison of the vector (by cosine similarity) is incorrect because vectors of the same length are required.
This is the related code:
lda_model_bow = models.LdaModel(corpus=bow_corpus, id2word=dictionary, num_topics=3, passes=1, random_state=47)
#---------------Calculating and Viewing the topics----------------------------
vec_bows = [dictionary.doc2bow(filtered_text.split()) for filtered_text in filtered_texts]
vec_lda_topics=[lda_model_bow[vec_bow] for vec_bow in vec_bows]
for id,vec_lda_topic in enumerate(vec_lda_topics):
print ('document ' ,id, 'topics: ', vec_lda_topic)
The output vectors is:
document 0 topics: [(1, 0.25697246), (2, 0.08026043), (3, 0.65391296)]
document 1 topics: [(2, 0.93666667)]
document 2 topics: [(2, 0.07910537), (3, 0.20132676)]
.....
As you can see, each vector has a different length, so it is not possible to perform cosine similarity between them.
I would like the output to be:
document 0 topics: [(1, 0.25697246), (2, 0.08026043), (3, 0.65391296)]
document 1 topics: [(1, 0.0), (2, 0.93666667), (3, 0.0)]
document 2 topics: [(1, 0.0), (2, 0.07910537), (3, 0.20132676)]
.....
Any ideas how to do it? tnx
python gensim lda topic-modeling cosine-similarity
python gensim lda topic-modeling cosine-similarity
asked Nov 21 '18 at 17:02


MatanMatan
879
879
add a comment |
add a comment |
2 Answers
2
active
oldest
votes
I have used gensim
for topic modeling before and I had not faced this issue. Ideally, if you pass num_topics=3
then it returns top 3 topics with the highest probability for each document. And then you should be able to generate the cosine similarity matrix by doing something like this:
lda_model_bow = models.LdaModel(corpus=bow_corpus, id2word=dictionary, num_topics=3, passes=1, random_state=47)
vec_lda_topics = lda_model_bow[bow_corpus]
sim_matrix = similarities.MatrixSimilarity(vec_lda_topics)
But for some reason, if you are getting unequal number of topics you can assume a zero probability value for the remaining topics and include them in your vector when you calculate similarity.
P.s.: If you could provide a sample of your input documents, it would be easier to reproduce your output and look into it.
According to the documentation of gensim.ldamodel:num_topics (int, optional) – The number of requested latent topics to be extracted from the training corpus.
If so, this is the total number of topics to which I want to divide the text rather than the top 3.
– Matan
Nov 21 '18 at 17:35
1
Did you also check this parameter:minimum_probability (float, optional)
– Topics with a probability lower than this threshold will be filtered out? Its default value is0.01
. It's possible that some of your topics are getting filtered out because of low probability.
– panktijk
Nov 21 '18 at 18:21
I just found this solution and wanted to update here. That's exactly the solution I was looking for. Thank you
– Matan
Nov 21 '18 at 18:36
add a comment |
So as panktijk says in the comment and also this topic , the solution is to cange minimum_probability
from the default value of 0.01
to 0.0
.
add a comment |
Your Answer
StackExchange.ifUsing("editor", function () {
StackExchange.using("externalEditor", function () {
StackExchange.using("snippets", function () {
StackExchange.snippets.init();
});
});
}, "code-snippets");
StackExchange.ready(function() {
var channelOptions = {
tags: "".split(" "),
id: "1"
};
initTagRenderer("".split(" "), "".split(" "), channelOptions);
StackExchange.using("externalEditor", function() {
// Have to fire editor after snippets, if snippets enabled
if (StackExchange.settings.snippets.snippetsEnabled) {
StackExchange.using("snippets", function() {
createEditor();
});
}
else {
createEditor();
}
});
function createEditor() {
StackExchange.prepareEditor({
heartbeatType: 'answer',
autoActivateHeartbeat: false,
convertImagesToLinks: true,
noModals: true,
showLowRepImageUploadWarning: true,
reputationToPostImages: 10,
bindNavPrevention: true,
postfix: "",
imageUploader: {
brandingHtml: "Powered by u003ca class="icon-imgur-white" href="https://imgur.com/"u003eu003c/au003e",
contentPolicyHtml: "User contributions licensed under u003ca href="https://creativecommons.org/licenses/by-sa/3.0/"u003ecc by-sa 3.0 with attribution requiredu003c/au003e u003ca href="https://stackoverflow.com/legal/content-policy"u003e(content policy)u003c/au003e",
allowUrls: true
},
onDemand: true,
discardSelector: ".discard-answer"
,immediatelyShowMarkdownHelp:true
});
}
});
Sign up or log in
StackExchange.ready(function () {
StackExchange.helpers.onClickDraftSave('#login-link');
});
Sign up using Google
Sign up using Facebook
Sign up using Email and Password
Post as a guest
Required, but never shown
StackExchange.ready(
function () {
StackExchange.openid.initPostLogin('.new-post-login', 'https%3a%2f%2fstackoverflow.com%2fquestions%2f53417171%2ffixed-size-topics-vector-in-gensim-lda-topic-modelling-for-finding-similar-texts%23new-answer', 'question_page');
}
);
Post as a guest
Required, but never shown
2 Answers
2
active
oldest
votes
2 Answers
2
active
oldest
votes
active
oldest
votes
active
oldest
votes
I have used gensim
for topic modeling before and I had not faced this issue. Ideally, if you pass num_topics=3
then it returns top 3 topics with the highest probability for each document. And then you should be able to generate the cosine similarity matrix by doing something like this:
lda_model_bow = models.LdaModel(corpus=bow_corpus, id2word=dictionary, num_topics=3, passes=1, random_state=47)
vec_lda_topics = lda_model_bow[bow_corpus]
sim_matrix = similarities.MatrixSimilarity(vec_lda_topics)
But for some reason, if you are getting unequal number of topics you can assume a zero probability value for the remaining topics and include them in your vector when you calculate similarity.
P.s.: If you could provide a sample of your input documents, it would be easier to reproduce your output and look into it.
According to the documentation of gensim.ldamodel:num_topics (int, optional) – The number of requested latent topics to be extracted from the training corpus.
If so, this is the total number of topics to which I want to divide the text rather than the top 3.
– Matan
Nov 21 '18 at 17:35
1
Did you also check this parameter:minimum_probability (float, optional)
– Topics with a probability lower than this threshold will be filtered out? Its default value is0.01
. It's possible that some of your topics are getting filtered out because of low probability.
– panktijk
Nov 21 '18 at 18:21
I just found this solution and wanted to update here. That's exactly the solution I was looking for. Thank you
– Matan
Nov 21 '18 at 18:36
add a comment |
I have used gensim
for topic modeling before and I had not faced this issue. Ideally, if you pass num_topics=3
then it returns top 3 topics with the highest probability for each document. And then you should be able to generate the cosine similarity matrix by doing something like this:
lda_model_bow = models.LdaModel(corpus=bow_corpus, id2word=dictionary, num_topics=3, passes=1, random_state=47)
vec_lda_topics = lda_model_bow[bow_corpus]
sim_matrix = similarities.MatrixSimilarity(vec_lda_topics)
But for some reason, if you are getting unequal number of topics you can assume a zero probability value for the remaining topics and include them in your vector when you calculate similarity.
P.s.: If you could provide a sample of your input documents, it would be easier to reproduce your output and look into it.
According to the documentation of gensim.ldamodel:num_topics (int, optional) – The number of requested latent topics to be extracted from the training corpus.
If so, this is the total number of topics to which I want to divide the text rather than the top 3.
– Matan
Nov 21 '18 at 17:35
1
Did you also check this parameter:minimum_probability (float, optional)
– Topics with a probability lower than this threshold will be filtered out? Its default value is0.01
. It's possible that some of your topics are getting filtered out because of low probability.
– panktijk
Nov 21 '18 at 18:21
I just found this solution and wanted to update here. That's exactly the solution I was looking for. Thank you
– Matan
Nov 21 '18 at 18:36
add a comment |
I have used gensim
for topic modeling before and I had not faced this issue. Ideally, if you pass num_topics=3
then it returns top 3 topics with the highest probability for each document. And then you should be able to generate the cosine similarity matrix by doing something like this:
lda_model_bow = models.LdaModel(corpus=bow_corpus, id2word=dictionary, num_topics=3, passes=1, random_state=47)
vec_lda_topics = lda_model_bow[bow_corpus]
sim_matrix = similarities.MatrixSimilarity(vec_lda_topics)
But for some reason, if you are getting unequal number of topics you can assume a zero probability value for the remaining topics and include them in your vector when you calculate similarity.
P.s.: If you could provide a sample of your input documents, it would be easier to reproduce your output and look into it.
I have used gensim
for topic modeling before and I had not faced this issue. Ideally, if you pass num_topics=3
then it returns top 3 topics with the highest probability for each document. And then you should be able to generate the cosine similarity matrix by doing something like this:
lda_model_bow = models.LdaModel(corpus=bow_corpus, id2word=dictionary, num_topics=3, passes=1, random_state=47)
vec_lda_topics = lda_model_bow[bow_corpus]
sim_matrix = similarities.MatrixSimilarity(vec_lda_topics)
But for some reason, if you are getting unequal number of topics you can assume a zero probability value for the remaining topics and include them in your vector when you calculate similarity.
P.s.: If you could provide a sample of your input documents, it would be easier to reproduce your output and look into it.
answered Nov 21 '18 at 17:18
panktijkpanktijk
95628
95628
According to the documentation of gensim.ldamodel:num_topics (int, optional) – The number of requested latent topics to be extracted from the training corpus.
If so, this is the total number of topics to which I want to divide the text rather than the top 3.
– Matan
Nov 21 '18 at 17:35
1
Did you also check this parameter:minimum_probability (float, optional)
– Topics with a probability lower than this threshold will be filtered out? Its default value is0.01
. It's possible that some of your topics are getting filtered out because of low probability.
– panktijk
Nov 21 '18 at 18:21
I just found this solution and wanted to update here. That's exactly the solution I was looking for. Thank you
– Matan
Nov 21 '18 at 18:36
add a comment |
According to the documentation of gensim.ldamodel:num_topics (int, optional) – The number of requested latent topics to be extracted from the training corpus.
If so, this is the total number of topics to which I want to divide the text rather than the top 3.
– Matan
Nov 21 '18 at 17:35
1
Did you also check this parameter:minimum_probability (float, optional)
– Topics with a probability lower than this threshold will be filtered out? Its default value is0.01
. It's possible that some of your topics are getting filtered out because of low probability.
– panktijk
Nov 21 '18 at 18:21
I just found this solution and wanted to update here. That's exactly the solution I was looking for. Thank you
– Matan
Nov 21 '18 at 18:36
According to the documentation of gensim.ldamodel:
num_topics (int, optional) – The number of requested latent topics to be extracted from the training corpus.
If so, this is the total number of topics to which I want to divide the text rather than the top 3.– Matan
Nov 21 '18 at 17:35
According to the documentation of gensim.ldamodel:
num_topics (int, optional) – The number of requested latent topics to be extracted from the training corpus.
If so, this is the total number of topics to which I want to divide the text rather than the top 3.– Matan
Nov 21 '18 at 17:35
1
1
Did you also check this parameter:
minimum_probability (float, optional)
– Topics with a probability lower than this threshold will be filtered out? Its default value is 0.01
. It's possible that some of your topics are getting filtered out because of low probability.– panktijk
Nov 21 '18 at 18:21
Did you also check this parameter:
minimum_probability (float, optional)
– Topics with a probability lower than this threshold will be filtered out? Its default value is 0.01
. It's possible that some of your topics are getting filtered out because of low probability.– panktijk
Nov 21 '18 at 18:21
I just found this solution and wanted to update here. That's exactly the solution I was looking for. Thank you
– Matan
Nov 21 '18 at 18:36
I just found this solution and wanted to update here. That's exactly the solution I was looking for. Thank you
– Matan
Nov 21 '18 at 18:36
add a comment |
So as panktijk says in the comment and also this topic , the solution is to cange minimum_probability
from the default value of 0.01
to 0.0
.
add a comment |
So as panktijk says in the comment and also this topic , the solution is to cange minimum_probability
from the default value of 0.01
to 0.0
.
add a comment |
So as panktijk says in the comment and also this topic , the solution is to cange minimum_probability
from the default value of 0.01
to 0.0
.
So as panktijk says in the comment and also this topic , the solution is to cange minimum_probability
from the default value of 0.01
to 0.0
.
answered Nov 21 '18 at 19:02


MatanMatan
879
879
add a comment |
add a comment |
Thanks for contributing an answer to Stack Overflow!
- Please be sure to answer the question. Provide details and share your research!
But avoid …
- Asking for help, clarification, or responding to other answers.
- Making statements based on opinion; back them up with references or personal experience.
To learn more, see our tips on writing great answers.
Sign up or log in
StackExchange.ready(function () {
StackExchange.helpers.onClickDraftSave('#login-link');
});
Sign up using Google
Sign up using Facebook
Sign up using Email and Password
Post as a guest
Required, but never shown
StackExchange.ready(
function () {
StackExchange.openid.initPostLogin('.new-post-login', 'https%3a%2f%2fstackoverflow.com%2fquestions%2f53417171%2ffixed-size-topics-vector-in-gensim-lda-topic-modelling-for-finding-similar-texts%23new-answer', 'question_page');
}
);
Post as a guest
Required, but never shown
Sign up or log in
StackExchange.ready(function () {
StackExchange.helpers.onClickDraftSave('#login-link');
});
Sign up using Google
Sign up using Facebook
Sign up using Email and Password
Post as a guest
Required, but never shown
Sign up or log in
StackExchange.ready(function () {
StackExchange.helpers.onClickDraftSave('#login-link');
});
Sign up using Google
Sign up using Facebook
Sign up using Email and Password
Post as a guest
Required, but never shown
Sign up or log in
StackExchange.ready(function () {
StackExchange.helpers.onClickDraftSave('#login-link');
});
Sign up using Google
Sign up using Facebook
Sign up using Email and Password
Sign up using Google
Sign up using Facebook
Sign up using Email and Password
Post as a guest
Required, but never shown
Required, but never shown
Required, but never shown
Required, but never shown
Required, but never shown
Required, but never shown
Required, but never shown
Required, but never shown
Required, but never shown
vq,0zebpgK,UP1JI6S9Qt4uqKY